Fundus blood vessel image segmentation method based on self-supervised learning
A supervised learning, blood vessel image technology, applied in the field of fundus blood vessel image segmentation based on self-supervised learning, can solve the problems of low contrast of eye images of blood vessel structures, inaccuracy of labeled samples, etc., and achieve the effect of improving segmentation ability and accurate pathological basis.
- Summary
- Abstract
- Description
- Claims
- Application Information
AI Technical Summary
Problems solved by technology
Method used
Image
Examples
Embodiment Construction
[0043] Below in conjunction with accompanying drawing, the present invention is described in further detail:
[0044] A kind of fundus blood vessel image segmentation method based on self-supervised learning provided by the present invention comprises the following steps:
[0045] S1. Set up a data set, which includes a labeled image sample D and unlabeled left and right eyeball image information X of the same patient T∈{L,R} , where D∈R C×H×W (Y i ,X i ), where, when T=L, X L represents the left eye image of the patient, when T=R, X R represents the right eye image of the patient, R C×H×W (Y i ,X i ) represents the right-eye image of the Cth image channel, H and W represent the length and width of the image, (Y i ,X i ) represents the i-th pixel of the C-th image and the length and width of the image are H and W; among them, the labeled image sample D refers to the image after the expert segmentation process, and has segmentation label information, each image Corres...
PUM
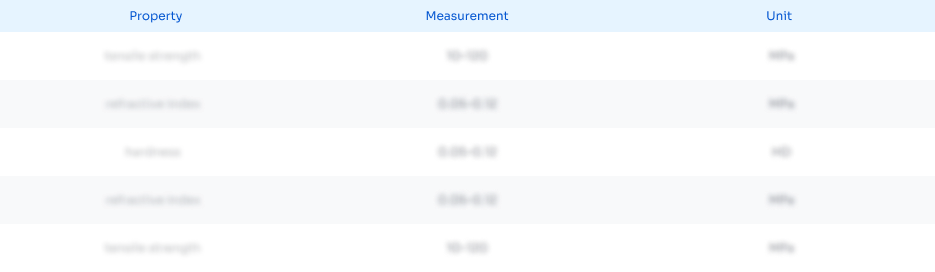
Abstract
Description
Claims
Application Information
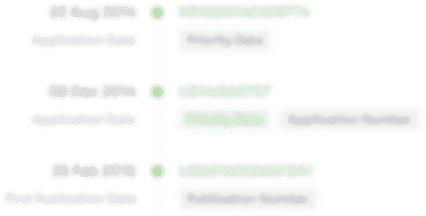
- R&D Engineer
- R&D Manager
- IP Professional
- Industry Leading Data Capabilities
- Powerful AI technology
- Patent DNA Extraction
Browse by: Latest US Patents, China's latest patents, Technical Efficacy Thesaurus, Application Domain, Technology Topic, Popular Technical Reports.
© 2024 PatSnap. All rights reserved.Legal|Privacy policy|Modern Slavery Act Transparency Statement|Sitemap|About US| Contact US: help@patsnap.com