Information diffusion prediction method based on time sequence hypergraph attention neural network
A neural network and information diffusion technology, which is applied in the field of information diffusion prediction based on time series hypergraph attention neural network, can solve the problems such as the inability to accurately capture the difference characteristics of users' neighbors in social networks, and the inability to describe the dynamic behavior of information diffusion. Achieving the effect of step-by-step forecasting
- Summary
- Abstract
- Description
- Claims
- Application Information
AI Technical Summary
Problems solved by technology
Method used
Image
Examples
Embodiment Construction
[0052] The present invention is described in further detail below in conjunction with accompanying drawing:
[0053] Aiming at the problems existing in the current information dissemination prediction task, the present invention jointly learns the user's preference from two aspects of the user's static friendship network and dynamic interaction network to predict information dissemination. Among them, the method not only utilizes the graph convolutional neural network to capture the user's static dependencies from the user's friendship network, but also innovatively designs a hypergraph attention network to dynamically learn users from the serialized information diffusion hypergraph. Interactions at the cascade level, and connections between cascades. At the same time, according to the cascaded features to be predicted, the embedding search module finds the vector corresponding to the user from the obtained two aspects of the user representation vector, so as to carry out the ...
PUM
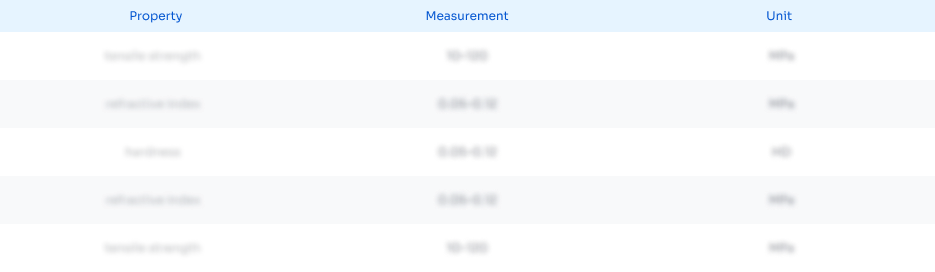
Abstract
Description
Claims
Application Information
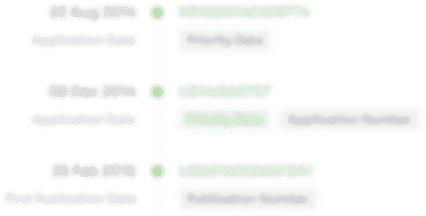
- R&D Engineer
- R&D Manager
- IP Professional
- Industry Leading Data Capabilities
- Powerful AI technology
- Patent DNA Extraction
Browse by: Latest US Patents, China's latest patents, Technical Efficacy Thesaurus, Application Domain, Technology Topic, Popular Technical Reports.
© 2024 PatSnap. All rights reserved.Legal|Privacy policy|Modern Slavery Act Transparency Statement|Sitemap|About US| Contact US: help@patsnap.com