Short-term irradiance prediction method and system based on historical data analysis
A technology of historical data and forecasting methods, applied in the field of short-term irradiance forecasting methods and forecasting systems, can solve problems such as heavy workload and complicated forecasting steps, and achieve the effect of improving forecasting accuracy
- Summary
- Abstract
- Description
- Claims
- Application Information
AI Technical Summary
Problems solved by technology
Method used
Image
Examples
Embodiment 1
[0071] The short-term irradiance prediction method based on historical data analysis of this embodiment, such as Figure 11 shown, including the following steps:
[0072] S10, historical data acquisition,
[0073] Obtain the historical solar irradiance data of the target area for one year;
[0074] S20, data preprocessing,
[0075] Firstly, the solar irradiance data obtained by S10 are divided into four types of seasonal data series: spring, summer, autumn and winter according to their time periods. Among them, the data from March to May are divided into spring data series; The data is divided into summer data series; the data from September to November is divided into autumn data series; the data series from December to February is divided into winter data series;
[0076] Then according to the irradiance of the solar irradiance data acquired by S10, all kinds of seasonal data series are divided into three types of weather data series: sunny day, cloudy day and rainy day, ...
Embodiment 2
[0117] The short-term irradiance prediction method based on historical data analysis of this embodiment, such as Figure 11 shown, including the following steps:
[0118] S10, historical data acquisition,
[0119] Obtain the historical solar irradiance data of the target area for one year;
[0120] S20, data preprocessing,
[0121] Firstly, the solar irradiance data obtained by S10 are divided into four types of seasonal data series: spring, summer, autumn and winter according to their time periods. Among them, the data from March to May are divided into spring data series; The data is divided into summer data series; the data from September to November is divided into autumn data series; the data series from December to February is divided into winter data series;
[0122] Then, according to the irradiance of the solar irradiance data acquired by S10, all kinds of seasonal data sequences are divided into three types of weather data sequences: sunny day, cloudy day and rain...
Embodiment 3
[0168] This embodiment utilizes the method of embodiment 2 to predict the weather on a certain day in a certain area, classify the spring irradiance in a certain area, and use the total 20 days of the weather type data sequence corresponding to the forecast day, and the data sequence of 20×24h=480h as The LSTM training and test data predict the 24h solar irradiance of the target day, the first 19 days are the training data, and the last day is the test data, and are compared with the actual solar irradiance data sequence of the target day.
[0169] In the LSTM neural network of this embodiment, the number of hidden layer units n=96*3, the maximum number of iterations m=250 times, m 1 = 125, the initial learning rate r = 0.005, the learning drop factor is f = 0.2, and the gradient threshold g = 1.
[0170] Depend on Figure 7 It can be seen that the fluctuation range of the sunny type irradiance data series is smaller than that of the actual data series, and is similar to the ...
PUM
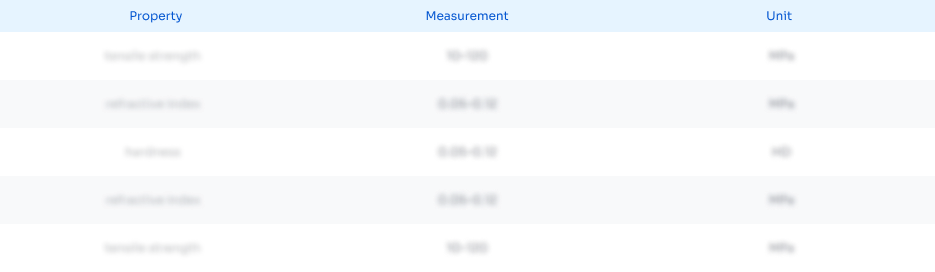
Abstract
Description
Claims
Application Information
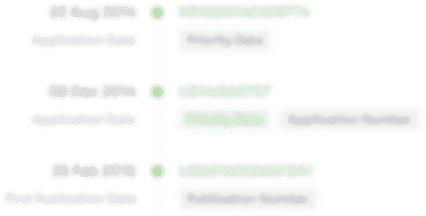
- R&D Engineer
- R&D Manager
- IP Professional
- Industry Leading Data Capabilities
- Powerful AI technology
- Patent DNA Extraction
Browse by: Latest US Patents, China's latest patents, Technical Efficacy Thesaurus, Application Domain, Technology Topic.
© 2024 PatSnap. All rights reserved.Legal|Privacy policy|Modern Slavery Act Transparency Statement|Sitemap