Semantic segmentation model training method and device, equipment and medium
A semantic segmentation and training method technology, applied in the computer field, can solve the problems of time-consuming and labor-intensive, increasing the pressure of data labeling, etc., to achieve the effect of reducing pressure, reducing dependence, and ensuring training effect
- Summary
- Abstract
- Description
- Claims
- Application Information
AI Technical Summary
Problems solved by technology
Method used
Image
Examples
Embodiment 1
[0035] figure 1 It is a flow chart of a semantic segmentation model training method provided in Embodiment 1 of the present invention. This embodiment is applicable to the situation of training a semantic segmentation model. The method can be executed by a semantic segmentation model training device, which can be realized by software and / or hardware, and integrated in electronic equipment. Such as figure 1 As shown, the method specifically includes the following steps:
[0036] S110. Based on the first sample image and the image category label corresponding to the first sample image, train the first semantic segmentation model.
[0037] Wherein, the first sample image may include a positive sample image and a negative sample image, the positive sample image may refer to a sample image in an application scene, and the negative sample image may be a sample image completely irrelevant to the application scene. For example, in an automatic driving scene, the scene image in the ...
Embodiment 2
[0055] figure 2 It is a flowchart of a semantic segmentation model training method provided by Embodiment 2 of the present invention. On the basis of the above-mentioned embodiments, this embodiment details the training process of the first semantic segmentation model and the second semantic segmentation model. describe. The explanations of terms that are the same as or corresponding to the above-mentioned embodiments will not be repeated here.
[0056] see figure 2 , the training method of the semantic segmentation model provided by the present embodiment has the following steps:
[0057] S210. Input the first sample image into the first semantic segmentation model, and determine the probability value that each first pixel in the first sample image is predicted to be each semantic label according to the output of the first semantic segmentation model, Wherein, the semantic labels include: a first semantic label identical to the image category label corresponding to the f...
Embodiment 3
[0090] Image 6 It is a flow chart of a training method for a semantic segmentation model provided by Embodiment 3 of the present invention. On the basis of the above-mentioned embodiments, this embodiment "uses the second semantic segmentation model after training as the target semantic segmentation model" Optimized. The explanations of terms that are the same as or corresponding to the above-mentioned embodiments will not be repeated here.
[0091] see Image 6 , the training method of the semantic segmentation model provided by the present embodiment has the following steps:
[0092] S610. Train the first semantic segmentation model based on the first sample image and the image category label corresponding to the first sample image.
[0093] S620. Input the second sample image into the first semantic segmentation model after training, and obtain the first pixel semantic label corresponding to the second sample image according to the output of the first semantic segmentat...
PUM
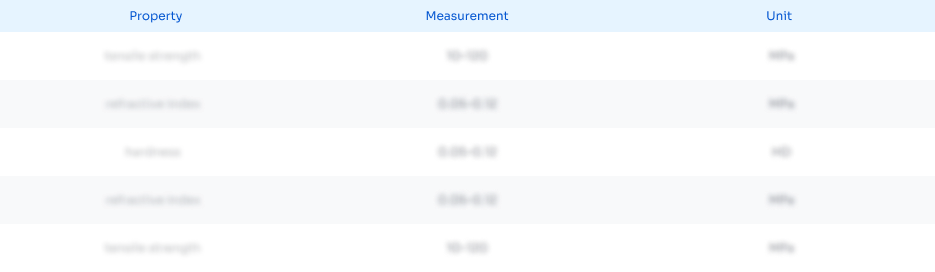
Abstract
Description
Claims
Application Information
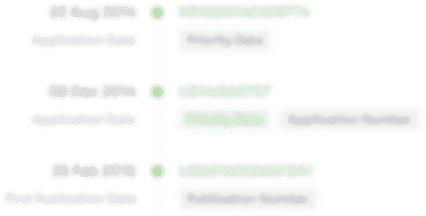
- R&D Engineer
- R&D Manager
- IP Professional
- Industry Leading Data Capabilities
- Powerful AI technology
- Patent DNA Extraction
Browse by: Latest US Patents, China's latest patents, Technical Efficacy Thesaurus, Application Domain, Technology Topic, Popular Technical Reports.
© 2024 PatSnap. All rights reserved.Legal|Privacy policy|Modern Slavery Act Transparency Statement|Sitemap|About US| Contact US: help@patsnap.com