Self-supervised tumor segmentation system based on layer decomposition
A layer decomposition and tumor technology, applied in the fields of computer vision and image processing, can solve the problem that the model cannot be directly applied to the downstream target task, and achieve the effect of improving the segmentation performance
- Summary
- Abstract
- Description
- Claims
- Application Information
AI Technical Summary
Problems solved by technology
Method used
Image
Examples
Embodiment 1
[0048] The present invention provides a self-supervised tumor segmentation system based on layer decomposition, such as figure 2 shown, including:
[0049] Module M1: Normal images are randomly generated 3D masks and transformed to obtain texture generation simulated tumor regions;
[0050] Module M2: fused the simulated tumor area with the normal image to obtain a synthesized tumor image;
[0051] Module M3: Train the deep convolutional neural network to learn layer decomposition, and synthesize the tumor image using the trained deep convolutional neural network to obtain the tumor segmentation map, restore the image of the tumor area, restore the normal image and synthesize the image, so that the input Tumor recognition features are extracted from tumor images for tumor segmentation.
[0052] The normal image is an image obtained by image acquisition of a healthy human body using a medical image acquisition device.
[0053] The self-supervised tumor segmentation method b...
PUM
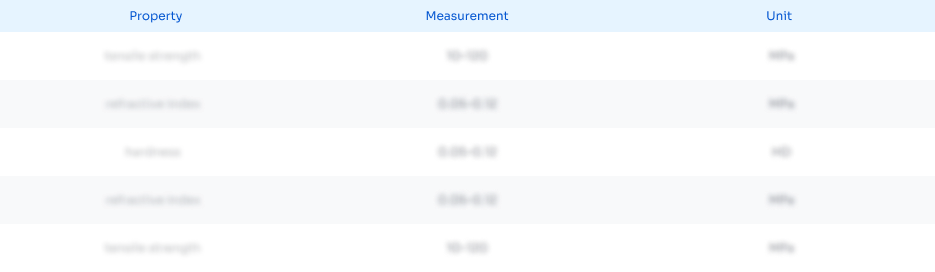
Abstract
Description
Claims
Application Information
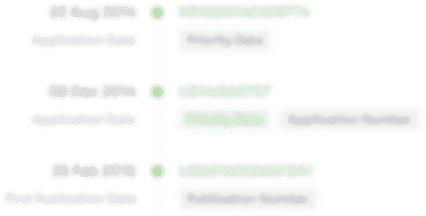
- R&D Engineer
- R&D Manager
- IP Professional
- Industry Leading Data Capabilities
- Powerful AI technology
- Patent DNA Extraction
Browse by: Latest US Patents, China's latest patents, Technical Efficacy Thesaurus, Application Domain, Technology Topic, Popular Technical Reports.
© 2024 PatSnap. All rights reserved.Legal|Privacy policy|Modern Slavery Act Transparency Statement|Sitemap|About US| Contact US: help@patsnap.com