ENSO diversity forecasting method based on artificial intelligence
An artificial intelligence and diversity technology, applied in weather condition forecasting, calculation models, instruments, etc., can solve the problem that the forecasting ability of the Central Pacific El Niño is not high, the spatial type of El Niño is not well resolved, and the spatial diversity cannot be displayed and other issues to achieve the effect of improving forecasting skills, reducing personnel and property losses, and breaking through forecasting bottlenecks
- Summary
- Abstract
- Description
- Claims
- Application Information
AI Technical Summary
Problems solved by technology
Method used
Image
Examples
Embodiment 1
[0023] This embodiment provides a method for forecasting ENSO diversity based on artificial intelligence, such as figure 1 shown, including the following steps:
[0024] S1. Using the EOF decomposition method, the first three main modes are extracted from the observed data of sea surface temperature anomalies in the equatorial Pacific Ocean: the zonal consistent type, the zonally inconsistent type, and the central warming type;
[0025] S2. Projecting the CMIP6 historical simulation data onto the three main modes EOF main mode, respectively obtaining three sets of historical simulation data PC values;
[0026] S3. Use the PC value of three sets of historical simulation data as the forecast value, and use the SSTA of the initial month and the two kinds of sea temperature data of the Tendency item as the input value of the training, and use the CMIP6 mode to train the improved deep learning model (VGG-11).
[0027] S4. Input the new observed data as the forecast input value int...
Embodiment 2
[0038] This embodiment provides a process of forecasting El Niño in the Central Pacific El Niño type occurrence area using the data of the equatorial Pacific Ocean surface from 1984 to 2017.
[0039] In this example, the warm pool index (WPI) is used to evaluate and compare the forecast results of the Central Pacific El Niño.
[0040] 1. Decompose the observed equatorial Pacific SSTA data using EOF to obtain the first three main modes (EOF1~3, such as figure 2 )
[0041] 2. Project the SSTA of 39 CMIP6 historical models (time: 1948–2014) into the three EOF main modes, and you can get the PC value of each month from 1948–2014; at the same time, you can get it from 39 historical models SSTA and Tendency items for each month.
[0042] 3. Use the initial month's SSTA and Tendency to input the deep learning model, and output 3 PC values forecasted N months in advance (N=1, 2, 3, 4, 5, 6, 7, 8, 9, 10, 11); Due to the large amount of data in 39 modes, after deep learning traini...
PUM
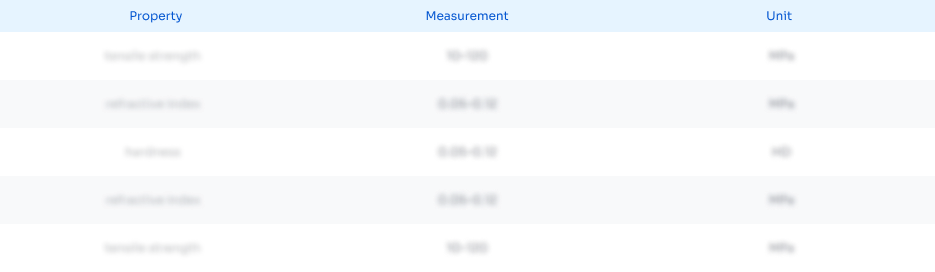
Abstract
Description
Claims
Application Information
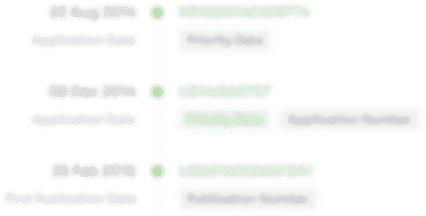
- R&D
- Intellectual Property
- Life Sciences
- Materials
- Tech Scout
- Unparalleled Data Quality
- Higher Quality Content
- 60% Fewer Hallucinations
Browse by: Latest US Patents, China's latest patents, Technical Efficacy Thesaurus, Application Domain, Technology Topic, Popular Technical Reports.
© 2025 PatSnap. All rights reserved.Legal|Privacy policy|Modern Slavery Act Transparency Statement|Sitemap|About US| Contact US: help@patsnap.com