Semi-supervised multi-label learning method based on SPUM data
A learning method and multi-label technology, applied in the field of semi-supervised multi-label learning based on SPUM data, can solve the problems of expensive, cumbersome and complete label data, and achieve the effect of reducing cost, maintaining performance and reducing communication burden
- Summary
- Abstract
- Description
- Claims
- Application Information
AI Technical Summary
Problems solved by technology
Method used
Image
Examples
Embodiment
[0075] We use mean average precision (mAP) as the main indicator for evaluating algorithm performance. In addition, in order to explore the performance of the algorithm under different labeling rates, we define an index LTR to express the ratio of labeled data to the total data, namely:
[0076] (12);
[0077] Each of the labeled data is multi-label data with a single positive example and no labels.
[0078] We first use a dataset with 5717 training images, 5823 test images and 20 class labels. Pascal Voc Experimental simulation is carried out on the data set. In order to show the effect of the event trigger strategy, each node when the number of training rounds is 1-100 is intercepted compared to The rate of change of and the triggering of each node event, that is, formula (10). The rate of change is shown in Figure 2, and Figure 3 is the In the case that each node satisfies the triggering condition of the event (10), we can get from the figure that as the number o...
PUM
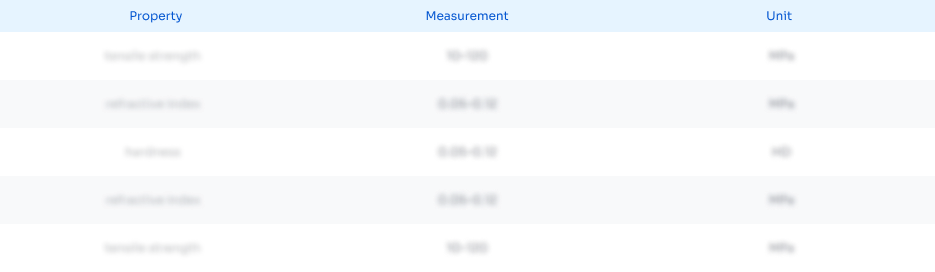
Abstract
Description
Claims
Application Information
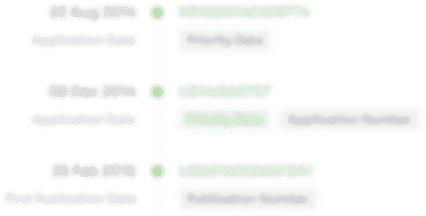
- R&D Engineer
- R&D Manager
- IP Professional
- Industry Leading Data Capabilities
- Powerful AI technology
- Patent DNA Extraction
Browse by: Latest US Patents, China's latest patents, Technical Efficacy Thesaurus, Application Domain, Technology Topic, Popular Technical Reports.
© 2024 PatSnap. All rights reserved.Legal|Privacy policy|Modern Slavery Act Transparency Statement|Sitemap|About US| Contact US: help@patsnap.com