Text-to-video cross-modal retrieval method based on multi-face video representation learning
A cross-modal, video technology, applied in video data retrieval, neural learning methods, video data query, etc., can solve problems such as not describing all the content, blurring multi-scene information, affecting the accuracy of text-video retrieval results, etc. To achieve the effect of improving the performance of retrieval
- Summary
- Abstract
- Description
- Claims
- Application Information
AI Technical Summary
Problems solved by technology
Method used
Image
Examples
Embodiment Construction
[0047] The present invention will be described in detail below with reference to the accompanying drawings and specific embodiments.
[0048] In order to solve the problem of text-to-video cross-modal retrieval, the present invention proposes a text-to-video cross-modal retrieval method based on multi-faceted video representation learning. In one embodiment, the specific steps are as follows:
[0049] (1) Using different feature extraction methods to extract the features of the two modalities of video and text, respectively.
[0050] (1-1) For a given video, it is pre-specified that j video frames are uniformly extracted from the video every 0.5 seconds. Deep features for each frame are then extracted using a convolutional neural network (CNN) model trained on the ImageNet dataset, such as the ResNet model. In this way, the video can be composed of a series of feature vectors {v 1 ,v 2 ,...,v t ,...,v j } to describe, where v t Represents the feature vector of the t-th f...
PUM
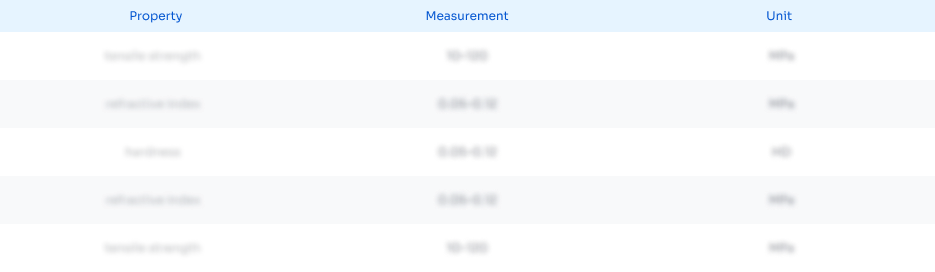
Abstract
Description
Claims
Application Information
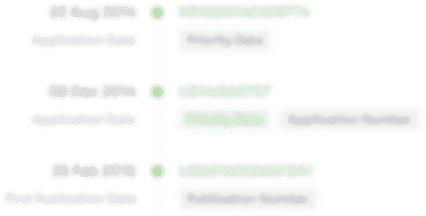
- R&D Engineer
- R&D Manager
- IP Professional
- Industry Leading Data Capabilities
- Powerful AI technology
- Patent DNA Extraction
Browse by: Latest US Patents, China's latest patents, Technical Efficacy Thesaurus, Application Domain, Technology Topic, Popular Technical Reports.
© 2024 PatSnap. All rights reserved.Legal|Privacy policy|Modern Slavery Act Transparency Statement|Sitemap|About US| Contact US: help@patsnap.com