A Deeply Supervised Cross-modal Adversarial Learning Method Based on Attention Mechanism
A learning method and attention technology, applied in the fields of multi-modal learning and information retrieval, can solve the problems of low semantic correlation and poor internal correlation, and achieve the effect of improving retrieval accuracy and good image-text mutual retrieval performance
- Summary
- Abstract
- Description
- Claims
- Application Information
AI Technical Summary
Problems solved by technology
Method used
Image
Examples
Embodiment Construction
[0055] The present invention will be further described in detail below with reference to the accompanying drawings and embodiments.
[0056] The deep-supervised cross-modal adversarial learning method based on the attention mechanism of the present invention, such as figure 1 , figure 2 shown, including the training process and retrieval process, as follows:
[0057] 1) Training process: Input the paired first-type objects, second-type objects and their class label information with the same semantics in the dataset D into the attention mechanism-based deep supervised adversarial network model for training, until the model Convergence to obtain the network model M. The first type of object is an image and the second type of object is text, or the first type of object is text and the second type of object is an image.
[0058] The training process is as follows:
[0059] 1.1) the data of the first type objects of different categories are input into the feature extraction ne...
PUM
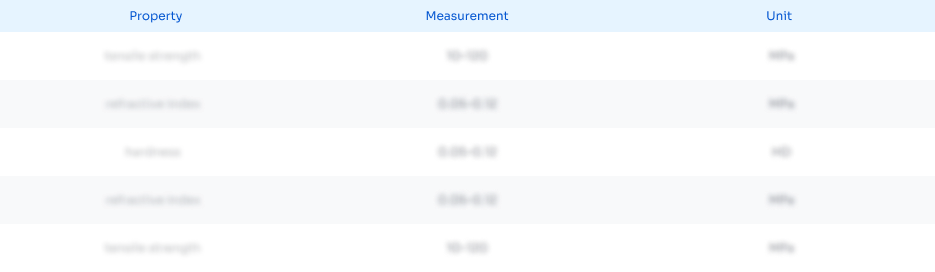
Abstract
Description
Claims
Application Information
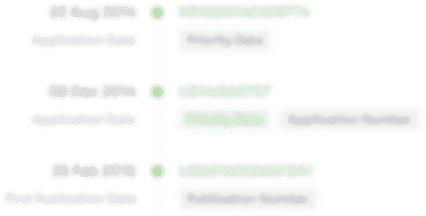
- R&D Engineer
- R&D Manager
- IP Professional
- Industry Leading Data Capabilities
- Powerful AI technology
- Patent DNA Extraction
Browse by: Latest US Patents, China's latest patents, Technical Efficacy Thesaurus, Application Domain, Technology Topic.
© 2024 PatSnap. All rights reserved.Legal|Privacy policy|Modern Slavery Act Transparency Statement|Sitemap