Unsupervised multi-source field adaptive method based on deep joint semantics
A multi-source field, unsupervised technology, applied in neural learning methods, instruments, biological neural network models, etc., can solve the problems of lack of category information and comprehensive consideration of context information, and achieve the effect of reducing cross-domain differences
- Summary
- Abstract
- Description
- Claims
- Application Information
AI Technical Summary
Problems solved by technology
Method used
Image
Examples
Embodiment 1
[0034] This example provides an unsupervised multi-source domain adaptive network based on deep learning for image classification, which specifically includes the following steps:
[0035] Step 1: Obtain training sample images from different sources;
[0036] To verify the performance of our proposed multi-source domain adaptation method, we select the Office-31 dataset as our sample images from the domain adaptation open competition and select 2 datasets as the source domain and the other dataset as the target area. The Office-31 dataset is a domain-adaptive benchmark dataset with 4110 images and three subsets with different distributions, each containing 31 categories: Amazon(A) downloaded from amazon.com website, Webcam(W) 795 web cameras, DSLR (D) 498 digital SLR cameras. In addition, office-31 is an unbalanced dataset, each domain contains a different number of images, three transfer tasks are implemented here: A, W→D; W, D→A; D, A→W are used for Test the performance o...
PUM
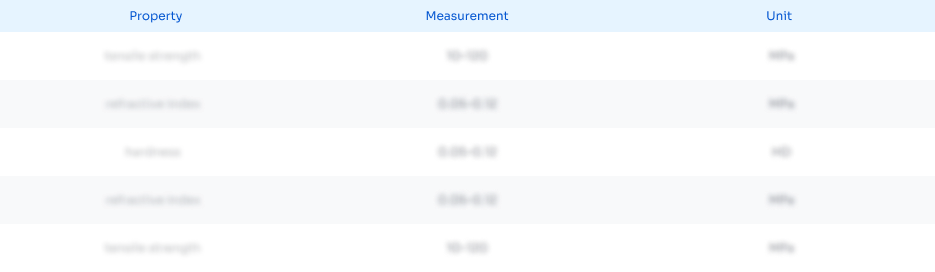
Abstract
Description
Claims
Application Information
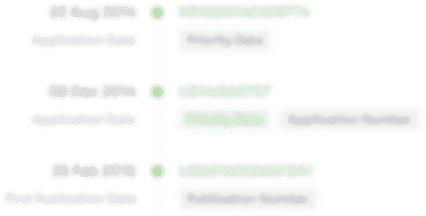
- R&D
- Intellectual Property
- Life Sciences
- Materials
- Tech Scout
- Unparalleled Data Quality
- Higher Quality Content
- 60% Fewer Hallucinations
Browse by: Latest US Patents, China's latest patents, Technical Efficacy Thesaurus, Application Domain, Technology Topic, Popular Technical Reports.
© 2025 PatSnap. All rights reserved.Legal|Privacy policy|Modern Slavery Act Transparency Statement|Sitemap|About US| Contact US: help@patsnap.com