Pattern recognition classification method expressed based on grouping sparsity
A sparse representation and pattern recognition technology, applied in the field of pattern recognition, can solve problems such as reducing the number of sample individuals, achieve the effect of enhancing sparsity, improving recognition ability, and reducing the amount of calculation
- Summary
- Abstract
- Description
- Claims
- Application Information
AI Technical Summary
Problems solved by technology
Method used
Image
Examples
Embodiment
[0068] An example of application of the invention is the problem of face recognition. The sample set is 2414 face images of 38 individuals in the Yale B extended database, with a size of 192×168 pixels. Each image is down-sampled to a size of 24×21 and arranged sequentially into a 504-dimensional vector. All face image vectors are randomly divided into two groups, one group is added to the training set, and the other group is added to the test set. Randomly select a vector from the test set, and use the above algorithm to obtain its grouped sparse representation on all samples in the training set. The obtained sparse solution is as follows: figure 2 As shown, l of the coefficient vector corresponding to each group 2 norm such as image 3 shown. It can be seen from the figure that the coefficient vector norm of the sixth group is the largest, and this sample is judged as the sixth person. The recognition rate obtained by cross-validation on the whole dataset is 94.2%.
PUM
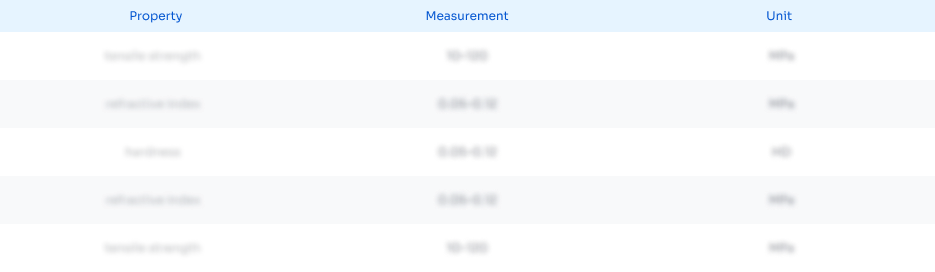
Abstract
Description
Claims
Application Information
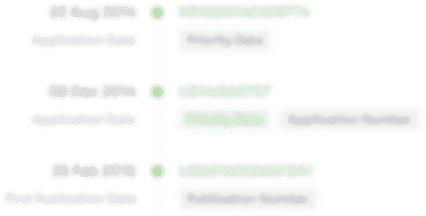
- R&D Engineer
- R&D Manager
- IP Professional
- Industry Leading Data Capabilities
- Powerful AI technology
- Patent DNA Extraction
Browse by: Latest US Patents, China's latest patents, Technical Efficacy Thesaurus, Application Domain, Technology Topic, Popular Technical Reports.
© 2024 PatSnap. All rights reserved.Legal|Privacy policy|Modern Slavery Act Transparency Statement|Sitemap|About US| Contact US: help@patsnap.com