Road traffic service level prediction method based on space-time characteristic aggregation
A service level and road traffic technology, applied in traffic flow detection, general control systems, instruments, etc., can solve problems such as large errors in road traffic status, inability to integrate multiple data, and spatial domain characteristics without time series characteristics. To achieve the effect of alleviating traffic pressure
- Summary
- Abstract
- Description
- Claims
- Application Information
AI Technical Summary
Problems solved by technology
Method used
Image
Examples
Embodiment Construction
[0035] The method of the present invention will be further described below through specific examples.
[0036] In this embodiment, the maximum entropy prediction model is used to predict a set of data from a certain road in Beijing on May 19, 2008, and compare and analyze it with the real road service level data to analyze the accuracy of the method.
[0037] First generate the training set of the maximum entropy prediction model. The training set used in this embodiment is the real road traffic data in Beijing. The training set includes the time and space characteristics of the road and the characteristics of the road itself, such as the number of lanes.
[0038] Observation feature data (o) is substituted into feature function f k (o, l i ) and generate a training set, because it is implemented through a program, so when generating a training set, the data must be edited into a specific format.
[0039] In this embodiment, the following format is used:
[0040] 1_alFlow1_...
PUM
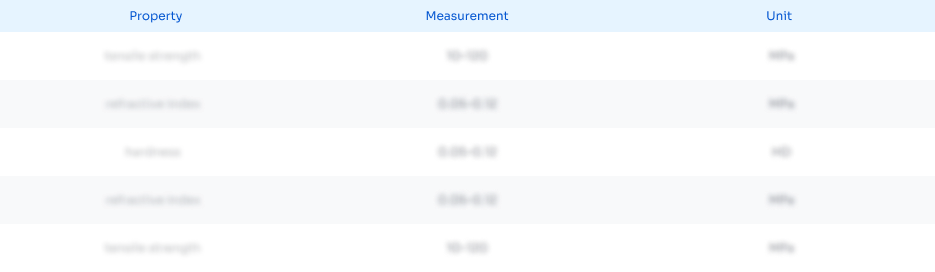
Abstract
Description
Claims
Application Information
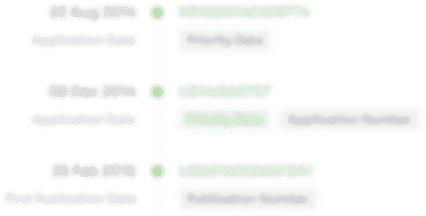
- R&D Engineer
- R&D Manager
- IP Professional
- Industry Leading Data Capabilities
- Powerful AI technology
- Patent DNA Extraction
Browse by: Latest US Patents, China's latest patents, Technical Efficacy Thesaurus, Application Domain, Technology Topic, Popular Technical Reports.
© 2024 PatSnap. All rights reserved.Legal|Privacy policy|Modern Slavery Act Transparency Statement|Sitemap|About US| Contact US: help@patsnap.com