Entropy sequencing-based semi-supervision spectral clustering method for determining clustering number
A sorting method and clustering number technology, which is applied in the fields of instruments, character and pattern recognition, computer parts, etc., can solve the problems of poor big data results, manual setting of thresholds, failures, etc., and achieve the effect of improving the clustering effect.
- Summary
- Abstract
- Description
- Claims
- Application Information
AI Technical Summary
Problems solved by technology
Method used
Image
Examples
Embodiment Construction
[0047] refer to figure 1 , the specific realization of the present invention comprises the following steps:
[0048] Step 1, calculate the scale parameter σ of each point i and the affinity matrix A of the dataset.
[0049] 1a) Input image dataset X={x 1 , x 2 ,...,x n}∈R d , where x i Represents any point in the data set, i∈(1,n), n is the number of data, and d represents the dimension of the data;
[0050] 1b) Calculate the scale parameter σ of each point of the input image data X i :
[0051] σ i = 1 m Σ d = 1 m | | x i - x d | |
[0052] Among them, σ i Represents the scale parameter of any point in the data points, x d is any point x in the data class X i The dth...
PUM
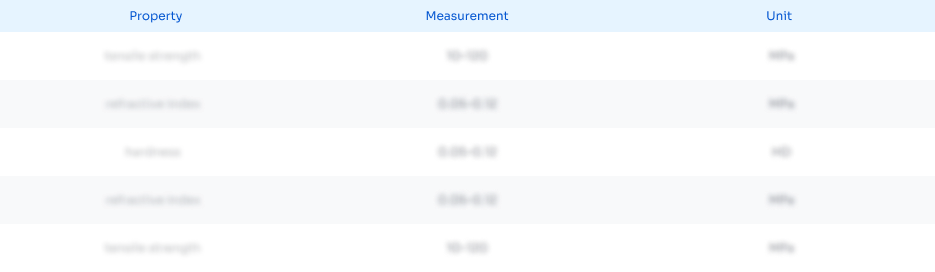
Abstract
Description
Claims
Application Information
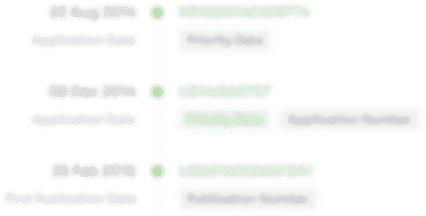
- R&D Engineer
- R&D Manager
- IP Professional
- Industry Leading Data Capabilities
- Powerful AI technology
- Patent DNA Extraction
Browse by: Latest US Patents, China's latest patents, Technical Efficacy Thesaurus, Application Domain, Technology Topic, Popular Technical Reports.
© 2024 PatSnap. All rights reserved.Legal|Privacy policy|Modern Slavery Act Transparency Statement|Sitemap|About US| Contact US: help@patsnap.com