Method for tracking multiple targets under unknown measurement noise distribution
An unknown measurement noise, multi-target tracking technology, applied in the field of target tracking, can solve the problems of reduced algorithm tracking performance, difficult-to-measure noise, affecting the speed of tracking, etc., saving computing time, high robustness and stability, and improving The effect of tracking speed
- Summary
- Abstract
- Description
- Claims
- Application Information
AI Technical Summary
Problems solved by technology
Method used
Image
Examples
Embodiment Construction
[0032] like figure 1 As shown, assuming that step 101 has been tracked, the state particle set at time k-1 is obtained as Then one-step target tracking requires 102 steps:
[0033] For the particle set at the previous moment (k-1), according to the formula (1) from the proposed distribution q k (·|x k-1 (i) ,Z k ) and p k (·|Z k Randomly select particles in ):
[0034] x ~ k ( i ) ~ q k ( · | x k - 1 ( i ) , ...
PUM
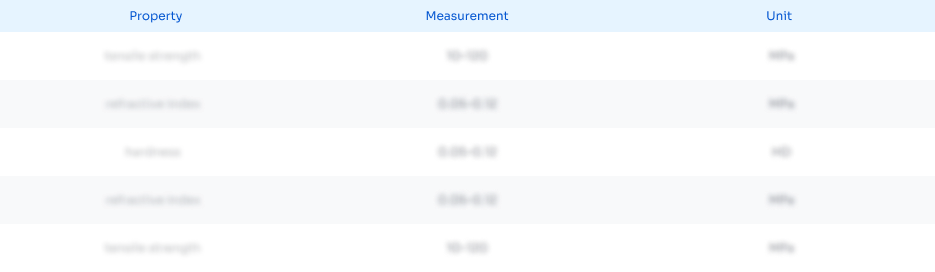
Abstract
Description
Claims
Application Information
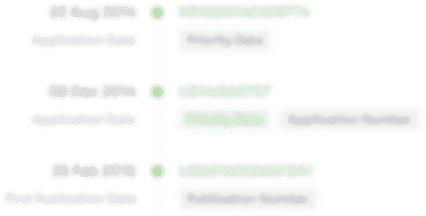
- R&D Engineer
- R&D Manager
- IP Professional
- Industry Leading Data Capabilities
- Powerful AI technology
- Patent DNA Extraction
Browse by: Latest US Patents, China's latest patents, Technical Efficacy Thesaurus, Application Domain, Technology Topic, Popular Technical Reports.
© 2024 PatSnap. All rights reserved.Legal|Privacy policy|Modern Slavery Act Transparency Statement|Sitemap|About US| Contact US: help@patsnap.com