Hyper-spectral image ground object recognition method based on sparse kernel representation (SKR)
A hyperspectral image and feature recognition technology, applied in character and pattern recognition, instruments, computer components, etc., can solve the problems of the influence of initial center point selection, low recognition rate, complicated training process, etc., to reduce time and Space complexity, improvement of recognition accuracy, effect of high recognition accuracy
- Summary
- Abstract
- Description
- Claims
- Application Information
AI Technical Summary
Problems solved by technology
Method used
Image
Examples
Embodiment Construction
[0023] refer to figure 1 , the specific implementation steps of the present invention are as follows:
[0024] Step 1: Select the real object category such as figure 2 The hyperspectral image shown builds the dictionary and test sample matrix, and normalizes them.
[0025] 1.1) The size of the hyperspectral image is 145×145, and there are 16 types of ground objects. Each pixel in the image can be regarded as a spectral vector sample composed of spectral information of 200 bands;
[0026] 1.2) In order to quantitatively illustrate the recognition accuracy of ground objects, all samples with known labels are selected for experimental simulation. Since the number of three types of ground objects, Alfalfa, Grass / pasture-mowed and Oats, is small, 15 are randomly selected in each category The sample is used as a training sample, and the remaining 13 types of ground objects have a large number. For each type, 50 samples are randomly selected as training samples, and all samples wi...
PUM
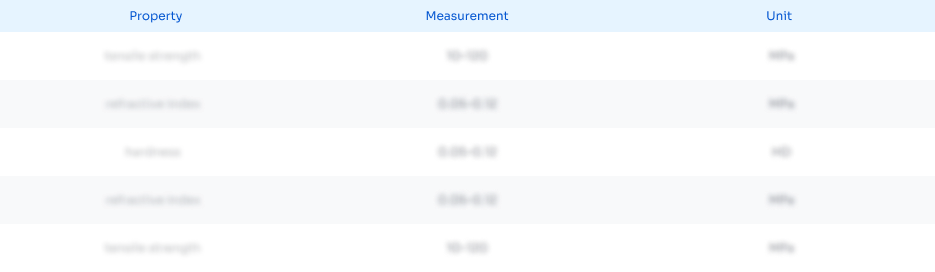
Abstract
Description
Claims
Application Information
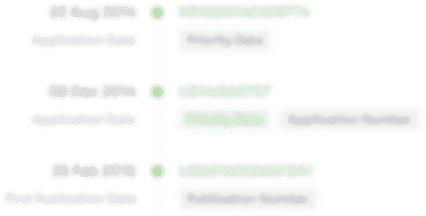
- R&D Engineer
- R&D Manager
- IP Professional
- Industry Leading Data Capabilities
- Powerful AI technology
- Patent DNA Extraction
Browse by: Latest US Patents, China's latest patents, Technical Efficacy Thesaurus, Application Domain, Technology Topic, Popular Technical Reports.
© 2024 PatSnap. All rights reserved.Legal|Privacy policy|Modern Slavery Act Transparency Statement|Sitemap|About US| Contact US: help@patsnap.com