Method for predicating remaining life of turbine engine based on degradation model matching
A turbine engine and degradation model technology, applied in special data processing applications, instruments, electrical digital data processing, etc., can solve the problem that the prediction effect cannot meet the prediction requirements, etc.
- Summary
- Abstract
- Description
- Claims
- Application Information
AI Technical Summary
Problems solved by technology
Method used
Image
Examples
specific Embodiment approach 1
[0014] Specific implementation mode one: combine figure 1 Describe this embodiment, the method for predicting the remaining life of a turbine engine based on the degradation model matching described in this embodiment, it includes specific steps as follows:
[0015] Step 1. Data preprocessing: Extract the variable that determines the operating state of the turbine engine from the collected raw data: c 1 is height, c 2 is the Mach number and c 3 is the throttle angle; the numerical values of the running state variables form a set c=(c 1 ,c 2 ,c 3 ) T ; c i means c at time t i Corresponding values of height, Mach number and throttle angle, c i =(c 1i , c 2i ,c 3i ) T , where i is a positive integer;
[0016] The values that can characterize the degradation process of the turbine engine are collected from 21 sensors located at different positions of the turbine engine, and the values form a 21-dimensional feature vector: x=(x 1 ,x 2 ,...,x 21 ) T , x i m...
specific Embodiment approach 2
[0028] Specific implementation mode two: combination figure 2 This embodiment is described. This embodiment is a further limitation of the method for predicting the remaining life of a turbine engine based on the degradation model matching described in Embodiment 1. The specific process of step 1 data preprocessing is as follows:
[0029] Step one one, to determine the set c=(c 1 ,c 2 ,c 3 ) T Use the K-means algorithm to cluster to obtain the p-type operating status Ω={O 1 ,O 2 ,...,O p}, p is a positive integer;
[0030] Step 12, divide the readings of the sensors in each extracted training entity according to the p-type operating status Ω, and divide them into p groups;
[0031] Step 13: Detect the change of the readings of each sensor in all training entities over time in each operating state, and select the sensor set Α={X 1 ,X 2 ,...,X m}, where m is a positive integer;
[0032] Step 14, a certain group of training entities divides the readings of the selecte...
specific Embodiment approach 3
[0042] Specific implementation mode three: combination figure 2 This embodiment is described. This embodiment is a further limitation of the method for predicting the remaining life of a turbine engine based on degradation model matching described in Embodiment 1. The specific process of establishing a degradation model library in step 2 is as follows:
[0043] Step 21. Divide the readings of the selected sensor set B according to the operating state Ω and divide them according to Perform linear regression to obtain the time series of health factors in group p;
[0044] Step 22. The p group of health factor time series is sorted by the time before the state division and restored to a complete set of health factor time series X 0 ={X 0 (k)|};
[0045] Step 23. Repeat steps 21 and 22 for all training entities, so that each training entity has a set of corresponding health factor time series;
[0046] Step two and four, using exponential regression model Fit the time seri...
PUM
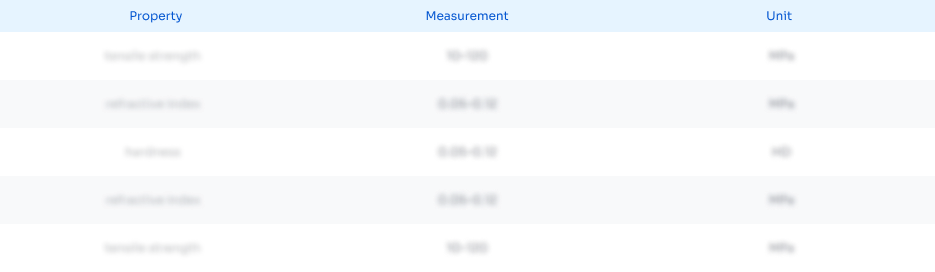
Abstract
Description
Claims
Application Information
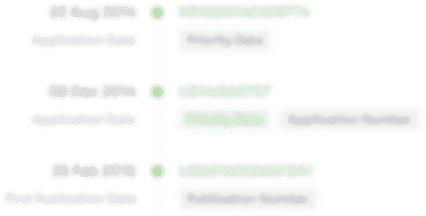
- R&D Engineer
- R&D Manager
- IP Professional
- Industry Leading Data Capabilities
- Powerful AI technology
- Patent DNA Extraction
Browse by: Latest US Patents, China's latest patents, Technical Efficacy Thesaurus, Application Domain, Technology Topic, Popular Technical Reports.
© 2024 PatSnap. All rights reserved.Legal|Privacy policy|Modern Slavery Act Transparency Statement|Sitemap|About US| Contact US: help@patsnap.com