Single-image super-resolution method based on multi-scale structural self-similarity and compressive sensing
A Structural Self-Similar, Compressed Sensing Technology
- Summary
- Abstract
- Description
- Claims
- Application Information
AI Technical Summary
Problems solved by technology
Method used
Image
Examples
Embodiment Construction
[0019] The present invention will be described in further detail below in conjunction with the accompanying drawings.
[0020] Let X ∈ R N Denotes a high-resolution image, Y ∈ R M represents a low-resolution image, Represents a high-resolution reconstructed image. Then the relationship between the high-resolution image X and the low-resolution image Y can be expressed as:
[0021] Y=DHX+υ (2.1)
[0022] Among them, D represents the downsampling matrix, H represents the fuzzy matrix, and υ represents the additive noise. The observation model shown in Equation (2.1) shows that the low-resolution image is obtained from the high-resolution image through blurring, down-sampling, and adding noise. The super-resolution method reconstructs high-resolution images by solving the inverse process of the degradation process, which can be expressed as the following optimization problem:
[0023] X ^ = arg min ...
PUM
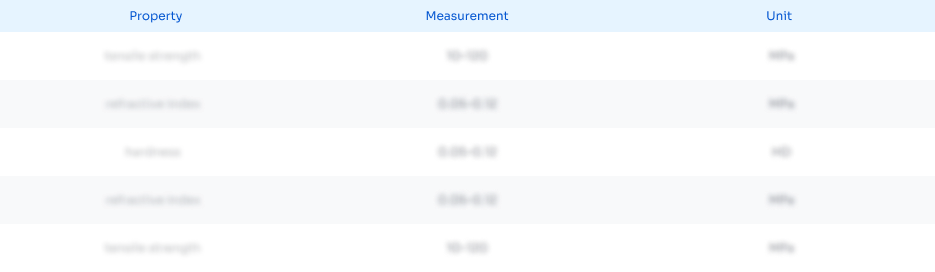
Abstract
Description
Claims
Application Information
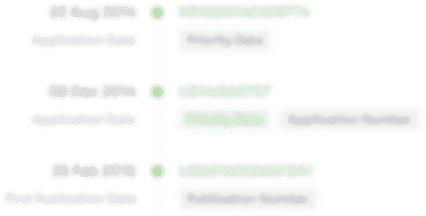
- R&D Engineer
- R&D Manager
- IP Professional
- Industry Leading Data Capabilities
- Powerful AI technology
- Patent DNA Extraction
Browse by: Latest US Patents, China's latest patents, Technical Efficacy Thesaurus, Application Domain, Technology Topic, Popular Technical Reports.
© 2024 PatSnap. All rights reserved.Legal|Privacy policy|Modern Slavery Act Transparency Statement|Sitemap|About US| Contact US: help@patsnap.com