Pattern classification method based on partial linear representation
A pattern classification and local linear technology, applied in the field of pattern recognition, can solve the problem of high time complexity of sparse coefficients, achieve the effect of reducing the number of training samples, reducing the difficulty of calculation, and improving the recognition rate
- Summary
- Abstract
- Description
- Claims
- Application Information
AI Technical Summary
Problems solved by technology
Method used
Image
Examples
Embodiment Construction
[0019] The technical scheme of invention is described in detail below:
[0020] Using the training sample set X containing c categories to identify the category to which the test sample y belongs includes the following steps.
[0021] X=[X 1 ,X 2 ,...,X c ],X i =[x i1 , x i2 ,...,x iNi ] represents the i-th class training sample set, X i contains N i samples, x ij ∈R d (R d Represents the d-dimensional real vector set) represents the jth training sample of the i-th class, y∈R d , c is a natural number, N i is a natural number.
[0022] Step 1, for the test sample y, construct its neighbor training sample set:
[0023] Step 1-1, calculate the test sample y to each training sample x in the training sample set X ij distance D ij .
[0024] D. ij =||y-x ij ||(i=1,2,...,c,j=1,2,...,N i );
[0025] Step 1-2, extract the first K nearest neighbor training samples x from the training sample set 1 ,x 2 ,...,x K , constituting the nearest neighbor training sampl...
PUM
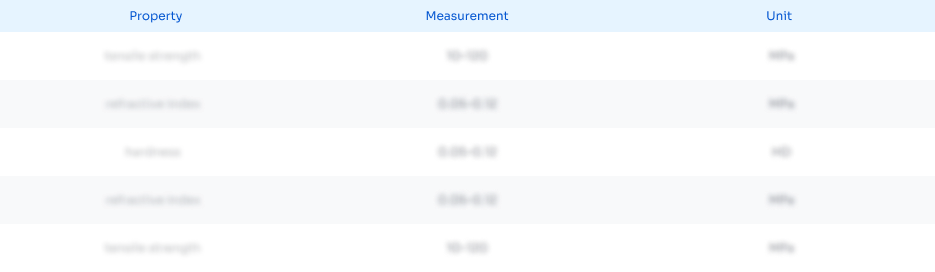
Abstract
Description
Claims
Application Information
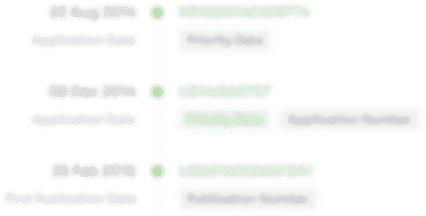
- Generate Ideas
- Intellectual Property
- Life Sciences
- Materials
- Tech Scout
- Unparalleled Data Quality
- Higher Quality Content
- 60% Fewer Hallucinations
Browse by: Latest US Patents, China's latest patents, Technical Efficacy Thesaurus, Application Domain, Technology Topic, Popular Technical Reports.
© 2025 PatSnap. All rights reserved.Legal|Privacy policy|Modern Slavery Act Transparency Statement|Sitemap|About US| Contact US: help@patsnap.com