Classification model training method combining active learning and transfer learning
A technology of transfer learning and classification models, which is applied in the field of efficient training of classification models by combining transfer learning and active learning, and can solve problems in areas where it is difficult to apply security and privacy, so as to avoid data security and privacy issues, learn efficiently, and avoid The effect of negative transfer
- Summary
- Abstract
- Description
- Claims
- Application Information
AI Technical Summary
Problems solved by technology
Method used
Image
Examples
Embodiment 1
[0072] In this embodiment, the technical solution provided by the present invention is applied to quickly train a personalized epileptic seizure detection model for an epileptic patient. The application background is as follows: the EEG signals of epileptic seizures vary greatly among different patients, and even the different seizure times of the same patient also vary greatly. The accumulation time and labeling cost of epileptic seizure labeling data are very high, and due to privacy protection reasons, it is difficult to obtain a large amount of other patient data from other hospitals or institutions. These two main reasons make it very difficult to train a general-purpose seizure detection model, and a more feasible solution is to train a personalized seizure detection model for each patient.
[0073] Preparation and instructions before implementing the program:
[0074] Source task: Onset and non-seizure binary classification of the epilepsy EEG dataset NEO.
[0075] So...
PUM
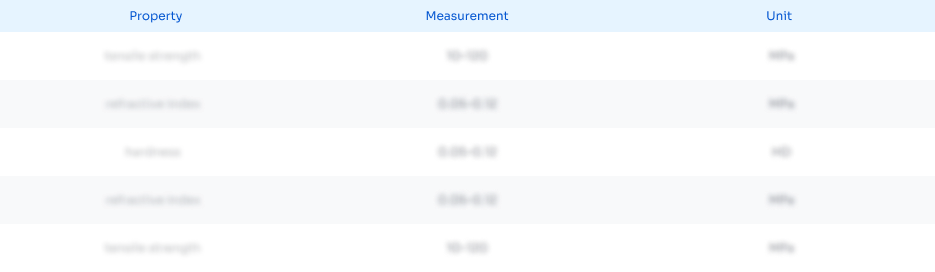
Abstract
Description
Claims
Application Information
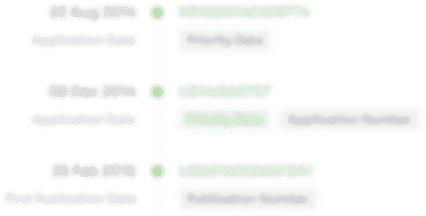
- R&D Engineer
- R&D Manager
- IP Professional
- Industry Leading Data Capabilities
- Powerful AI technology
- Patent DNA Extraction
Browse by: Latest US Patents, China's latest patents, Technical Efficacy Thesaurus, Application Domain, Technology Topic, Popular Technical Reports.
© 2024 PatSnap. All rights reserved.Legal|Privacy policy|Modern Slavery Act Transparency Statement|Sitemap|About US| Contact US: help@patsnap.com