Fault diagnosis method of rolling bearing under variable working conditions based on feature transfer learning
A rolling bearing and fault diagnosis technology, which is applied in the testing of mechanical components, testing of machine/structural components, instruments, etc., can solve the problems of low diagnostic accuracy and the inability to obtain a large amount of tagged vibration data for rolling bearings
- Summary
- Abstract
- Description
- Claims
- Application Information
AI Technical Summary
Problems solved by technology
Method used
Image
Examples
Embodiment Construction
[0081] Such as Figures 1 to 7 As shown, the realization process of the rolling bearing fault diagnosis method under variable working conditions based on feature transfer learning (based on feature multi-core SSTCA-SVM rolling bearing fault diagnosis method under variable working conditions) is given in this embodiment as follows:
[0082] (1) Feature extraction:
[0083] Perform VMD operation on the vibration signals of rolling bearings with known and unknown working conditions (normal under different speeds and different load conditions, different failure degrees of the inner ring, different failure degrees of the outer ring, and different failure degrees of the rolling elements), and use observation Determine the number of IMFs to be decomposed, build a matrix for the IMF, and perform SVD to obtain singular values, and at the same time obtain the singular value entropy; then extract the time domain and frequency domain characteristic indicators of the vibration signal;
[...
PUM
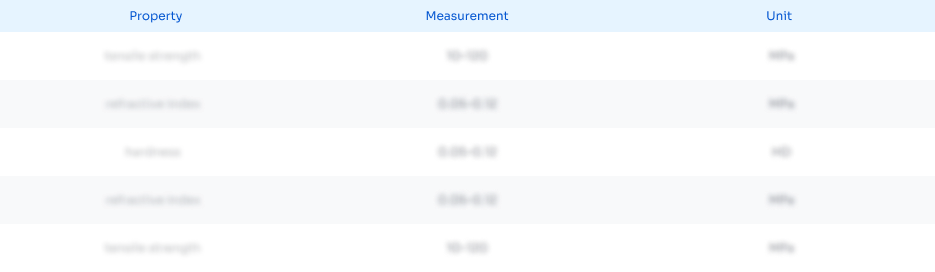
Abstract
Description
Claims
Application Information
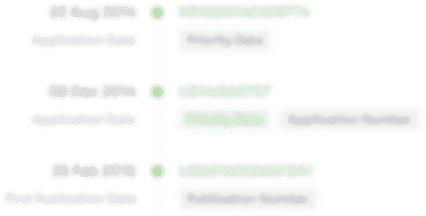
- R&D Engineer
- R&D Manager
- IP Professional
- Industry Leading Data Capabilities
- Powerful AI technology
- Patent DNA Extraction
Browse by: Latest US Patents, China's latest patents, Technical Efficacy Thesaurus, Application Domain, Technology Topic, Popular Technical Reports.
© 2024 PatSnap. All rights reserved.Legal|Privacy policy|Modern Slavery Act Transparency Statement|Sitemap|About US| Contact US: help@patsnap.com