A Navigation Path Planning Method Based on Policy Reuse and Reinforcement Learning
A navigation path and reinforcement learning technology, applied in navigation, mapping and navigation, navigation computing tools, etc., can solve the problem of insufficient source strategy reuse, and achieve the effect of rapid planning of navigation paths, accurate navigation tasks, and avoidance of negative transfer.
- Summary
- Abstract
- Description
- Claims
- Application Information
AI Technical Summary
Problems solved by technology
Method used
Image
Examples
specific Embodiment approach 1
[0031] Specific implementation mode one: a navigation path planning method based on policy reuse and reinforcement learning described in this implementation mode, the method includes the following steps:
[0032] Step 1. Select the strategy library corresponding to the current road network map, and calculate the important status of the source strategy that does not contain the key map position in the strategy library;
[0033] Step 2: Set the maximum number of training cycles to K (K can be set larger, and if the actual self-learning condition is reached, it will automatically jump out and no longer use the strategy), use the confidence to select the reuse strategy from the source strategy of the strategy library, and Reuse its own strategy or selected reuse strategy;
[0034] Step 3. The new policy obtained by policy reuse is updated through reinforcement learning to obtain an updated new policy;
[0035] Step 4: Determine whether to add the updated strategy to the strategy ...
specific Embodiment approach 2
[0036] Specific implementation mode two: the difference between this implementation mode and specific implementation mode one is: the specific process of the step one is:
[0037] Select the strategy library corresponding to the current road network map. For the source strategy that does not contain the key map position (important state) in the strategy library, you need to calculate the important state of the source strategy that does not include the key map position;
[0038] For any source policy that needs to calculate important states, initialize the floating threshold θ=0, and then enter M' (M'≥8, smaller) policy execution cycles, and select the road network map in the first step of each policy execution cycle An edge position of as the initial state s 0 (The first eight can take the edge positions of the eight directions of the road network map as the initial state), for the tth step of each strategy execution cycle, the current state of the vehicle navigation system is...
specific Embodiment approach 3
[0045] Specific implementation mode three: the difference between this implementation mode and specific implementation mode two is: the specific process of said step two is:
[0046] The selection of the source strategy considers the state value function of each strategy in the strategy library, and excludes some strategies in the strategy library from the alternative strategies based on the change of confidence in real time:
[0047] Step 21. In the first training cycle, each source strategy π k The initial confidence p k are set to 0.5; for each subsequent training cycle, each source strategy π k The confidence level of will be determined by whether the vehicle navigation system reached the target position s in the previous training cycle G and by source policy π k important state of To determine, as shown in formula (3):
[0048]
[0049] Among them: I k represent the conditions of judgment;
[0050] Let τ′ be a trajectory containing all the states passed in the ...
PUM
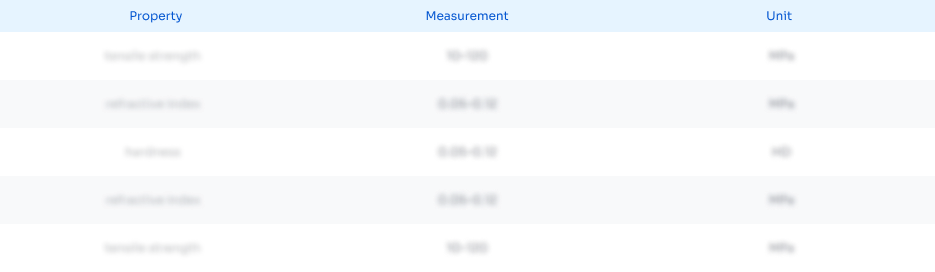
Abstract
Description
Claims
Application Information
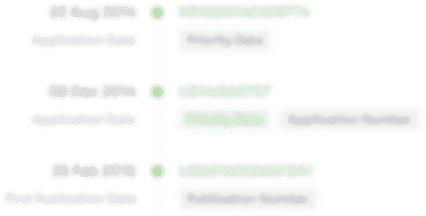
- R&D Engineer
- R&D Manager
- IP Professional
- Industry Leading Data Capabilities
- Powerful AI technology
- Patent DNA Extraction
Browse by: Latest US Patents, China's latest patents, Technical Efficacy Thesaurus, Application Domain, Technology Topic, Popular Technical Reports.
© 2024 PatSnap. All rights reserved.Legal|Privacy policy|Modern Slavery Act Transparency Statement|Sitemap|About US| Contact US: help@patsnap.com