Text categorization method based on probability word selection and supervision subject model
A topic model and text classification technology, applied in special data processing applications, instruments, electronic digital data processing, etc., can solve problems such as the inability to use word ambiguity, affecting the performance of topic models, and affecting the performance of topic models.
- Summary
- Abstract
- Description
- Claims
- Application Information
AI Technical Summary
Problems solved by technology
Method used
Image
Examples
Embodiment
[0172] From http: / / web.ist.utl.pt / ~acardoso / datasets / Downloaded training text 20 ng-train-all-terms and test text 20ng-test-all-terms, remove the text that appears no more than 3 words, get D tr =11285 training texts and D tr =8571 test texts. In the experiment, the number of topics K is set to 20, and other experimental parameters are selected as shown in Table 1:
[0173] Table 1
[0174]
[0175] For the training text, perform the following steps:
[0176] 1) Remove punctuation marks, count word frequency information and category information, and form a word list with a size of 73712 and a category list with a size of 20;
[0177] 2) Initialize the topic proportion vector α, the topic word matrix β, the topic word discrimination matrix ψ and the regression coefficient matrix η:
[0178] (2.1) For α, ψ and η, α k =0.1, ψ kv =0.5, η cv =0,k=1,...,K,c=1,...,C,v=1,...,V;
[0179] (2.2) For β, shilling k=1,...,K, v=1,...,V, where the rand function randomly genera...
PUM
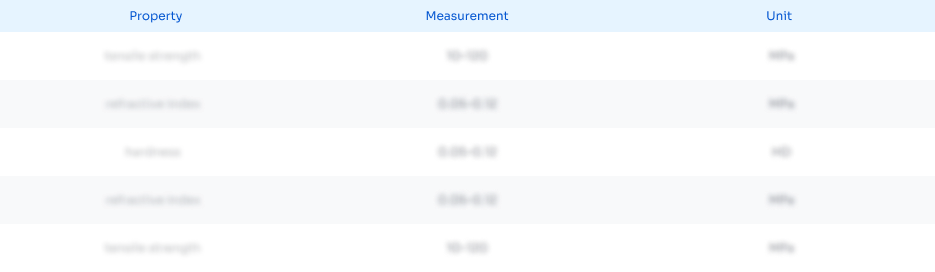
Abstract
Description
Claims
Application Information
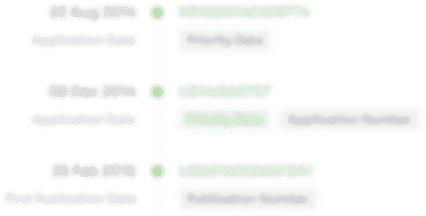
- R&D Engineer
- R&D Manager
- IP Professional
- Industry Leading Data Capabilities
- Powerful AI technology
- Patent DNA Extraction
Browse by: Latest US Patents, China's latest patents, Technical Efficacy Thesaurus, Application Domain, Technology Topic, Popular Technical Reports.
© 2024 PatSnap. All rights reserved.Legal|Privacy policy|Modern Slavery Act Transparency Statement|Sitemap|About US| Contact US: help@patsnap.com