Matrix decomposition recommendation method based on Bayesian probability with social relations and project content
A probability matrix decomposition and social relationship technology, applied in the field of Bayesian probability matrix decomposition recommendation, can solve the problem that the recommendation system cannot solve data sparseness or cold start well, so as to alleviate the problem of cold start, data sparseness, and good The effect of the cold start problem
- Summary
- Abstract
- Description
- Claims
- Application Information
AI Technical Summary
Problems solved by technology
Method used
Image
Examples
specific Embodiment approach
[0025] Step (1), using Probabilistic Matrix Factorization (PMF, Probabilistic Matrix Factorization) to conduct implicit matrix analysis on the observation evaluation matrix, to obtain the hidden user feature matrix and hidden item feature matrix:
[0026] Suppose the system has M users and N projects. Matrix R represents the observation evaluation matrix, R ij Indicates user i's rating for item j. U∈R M×D and V ∈ R N×D Denote the hidden user feature matrix and hidden item feature matrix respectively, where the row vector U i and V j represent latent feature vectors for users and items, respectively. The constant D is the dimension size of user feature vector and item feature vector and is much smaller than M and N. Suppose the conditional probability of observing the evaluation matrix R is as follows:
[0027] p ( R | U , V , σ ...
PUM
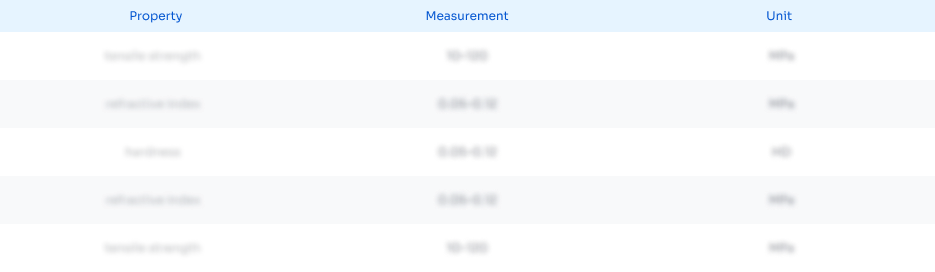
Abstract
Description
Claims
Application Information
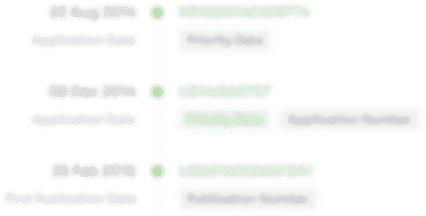
- R&D
- Intellectual Property
- Life Sciences
- Materials
- Tech Scout
- Unparalleled Data Quality
- Higher Quality Content
- 60% Fewer Hallucinations
Browse by: Latest US Patents, China's latest patents, Technical Efficacy Thesaurus, Application Domain, Technology Topic, Popular Technical Reports.
© 2025 PatSnap. All rights reserved.Legal|Privacy policy|Modern Slavery Act Transparency Statement|Sitemap|About US| Contact US: help@patsnap.com