Task scheduling algorithm based on MapReduce
A technology of task scheduling and scheduling algorithm, applied in the direction of program startup/switching, multiprogramming device, etc.
- Summary
- Abstract
- Description
- Claims
- Application Information
AI Technical Summary
Problems solved by technology
Method used
Image
Examples
Embodiment 1
[0069] A task scheduling algorithm based on MapReduce. In a heterogeneous cluster environment, the multi-task scheduling algorithm based on the ant colony algorithm measures the processing performance of the computing nodes, according to the new task target transfer function and the new node update rules, according to The local computing principle distributes tasks to individual computing nodes.
Embodiment 2
[0071] On the basis of Embodiment 1, the measurement of the processing performance of computing nodes described in this embodiment mainly measures the initial processing capability of the node and the target transition probability of tasks assigned to the node, wherein the initial processing capability of the node is based on the processing speed, Memory capacity, number of CPUs, and network transmission bandwidth are comprehensively measured, and thresholds are set for these four metrics. If the thresholds are exceeded, the thresholds are used for calculation; in task scheduling, a scheduler is set up to be responsible for Computes the initial transition probabilities of task assignments to requesting nodes.
Embodiment 3
[0073] On the basis of Embodiment 2, the initial processing capability of the node depends on the initial pheromone of the node, which is calculated and determined by formula 1.1.
PUM
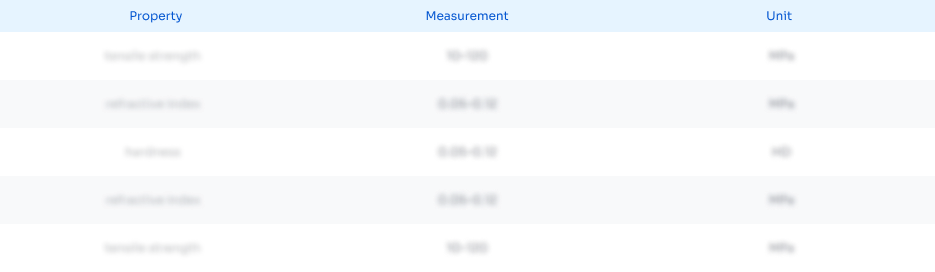
Abstract
Description
Claims
Application Information
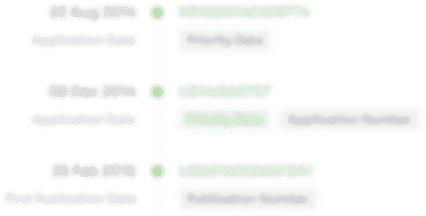
- R&D
- Intellectual Property
- Life Sciences
- Materials
- Tech Scout
- Unparalleled Data Quality
- Higher Quality Content
- 60% Fewer Hallucinations
Browse by: Latest US Patents, China's latest patents, Technical Efficacy Thesaurus, Application Domain, Technology Topic, Popular Technical Reports.
© 2025 PatSnap. All rights reserved.Legal|Privacy policy|Modern Slavery Act Transparency Statement|Sitemap|About US| Contact US: help@patsnap.com