A Method of Improving the Accuracy of Speech Recognition Based on the Number of Dynamic HMM Events
A technology of the number of events and the accuracy rate, applied in speech recognition, speech analysis, instruments, etc., can solve the problem of the decline of the recognition accuracy rate, and achieve the effect of improving the accuracy rate, improving the recognition accuracy rate, and improving the recognition accuracy rate.
- Summary
- Abstract
- Description
- Claims
- Application Information
AI Technical Summary
Problems solved by technology
Method used
Image
Examples
Embodiment Construction
[0016] The present invention will be further described below in conjunction with the accompanying drawings and specific embodiments.
[0017] The Hidden Markov HMM model probability parameter that the present invention uses is as follows:
[0018] (1) N, the number of events in the HMM model. The number of events in the HMM model is implicit. In the subsequent expressions, each event in the label model is {S 1 ,S 2 ,...,S N}, the event at time t is q t .
[0019] (2) M, the number of elements in the sequence that can be observed under each event in the HMM model, that is, the number of observed symbols. Mark each observation symbol as V={v 1 ,v 2 ,L,v M}, the observation sequence is O={o 1 ,o 2 ,L,o T}, where o t is an observation symbol in the set V, and T is the length of the observation sequence.
[0020] (3) Event transition probability distribution A=[a ij ],in
[0021] a ij =p[q t+1 = S j |q t = S i ] 1≤i≤N, 1≤j≤N.
[0022] (4) Observation sequence p...
PUM
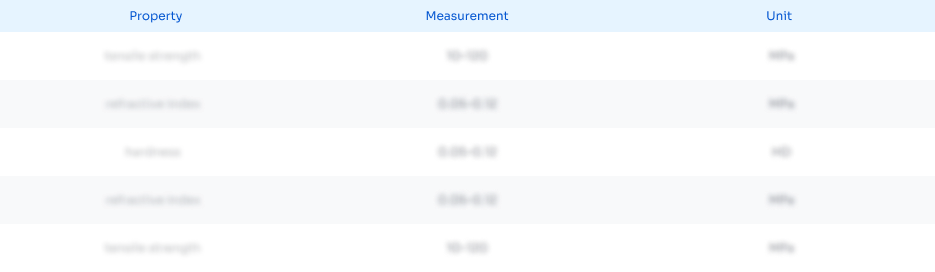
Abstract
Description
Claims
Application Information
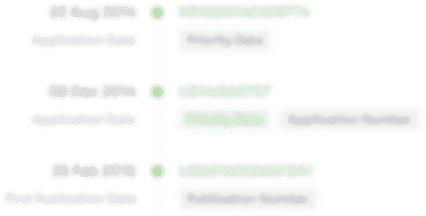
- R&D Engineer
- R&D Manager
- IP Professional
- Industry Leading Data Capabilities
- Powerful AI technology
- Patent DNA Extraction
Browse by: Latest US Patents, China's latest patents, Technical Efficacy Thesaurus, Application Domain, Technology Topic, Popular Technical Reports.
© 2024 PatSnap. All rights reserved.Legal|Privacy policy|Modern Slavery Act Transparency Statement|Sitemap|About US| Contact US: help@patsnap.com