Method and device for predicting advertisement click rate
An advertisement click and advertisement technology, applied in the field of big data computing, can solve the problems of occupying memory, data impact, missing, etc., and achieve the effect of improving utilization efficiency and accurate advertisement click rate.
- Summary
- Abstract
- Description
- Claims
- Application Information
AI Technical Summary
Problems solved by technology
Method used
Image
Examples
Embodiment 1
[0049] This method proposes an idea and its specific algorithmic process and expresses it with a formula. It can be more preparatory to the existing advertising click-through rate estimation method to obtain a more effective advertising click-through rate estimation value, which can Select the most valuable features, exclude irrelevant or less relevant features, and are not sensitive to the lack of data, and automatically select irrelevant features.
[0050] Such as figure 1 , 2 , 3, a method for predicting the click-through rate of an advertisement, including:
[0051] Step 1) Obtain historical data samples as training data;
[0052] Use the random forest method to select the most valuable features and exclude irrelevant or less relevant features;
[0053] Step 2) Using the regression model to predict the click-through rate of advertisements on the training data obtained above after removing irrelevant or less relevant features.
[0054] In step 1), obtain historical data...
Embodiment 2
[0062] Step 2) can select the existing technology, of course, can also be further optimized according to the present invention, specifically, step 2), use the regression model to carry out advertisement on the above-mentioned obtained data after removing irrelevant or less relevant features Forecasting the click-through rate, specifically including: forecasting based on the Logistic regression model;
[0063] P{y=1|f(x)}=1 / (1+exp(-(w x+b))), (1)
[0064] Among them, f(x) is the predicted value of the advertisement through logistic regression, w is the advertisement weight vector, x is the advertisement sample data after removing irrelevant or less relevant features, P{y=1|f(x)} Represents the posterior probability value that the advertisement is actually clicked by the user through the predicted value, so that the probability of a new advertisement sample being clicked is obtained through the above formula.
[0065] Further include: Step 22) By constructing a loss function, u...
Embodiment 3
[0070] The following are specific embodiments, specifically including:
[0071] 1. Use the random forest method to select the most valuable features, exclude irrelevant or less relevant features, not sensitive to the lack of data, and automatically select irrelevant features.
[0072] The details are as follows:
[0073] For the training data T={x,y}, x is the training sample, the number of samples is n, the feature dimension is m-dimensional, y is the label of the corresponding sample, 0 means that the advertisement is not clicked by the user, 1 means The advertisement is clicked by the user. Randomly sample n training sample data, the number is also n, and at the same time, randomly select m-dimensional features to obtain a d(
PUM
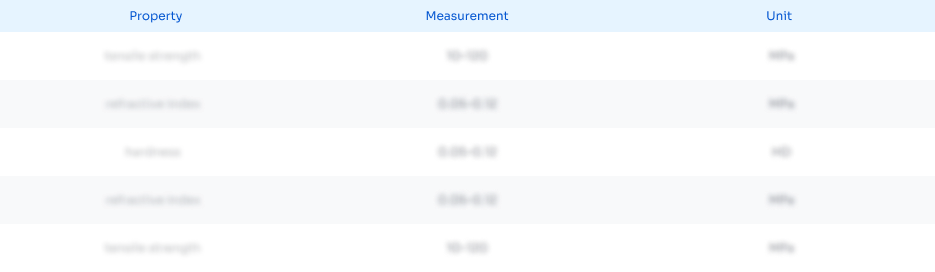
Abstract
Description
Claims
Application Information
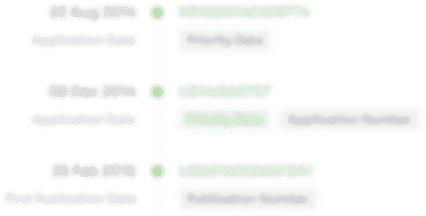
- R&D Engineer
- R&D Manager
- IP Professional
- Industry Leading Data Capabilities
- Powerful AI technology
- Patent DNA Extraction
Browse by: Latest US Patents, China's latest patents, Technical Efficacy Thesaurus, Application Domain, Technology Topic.
© 2024 PatSnap. All rights reserved.Legal|Privacy policy|Modern Slavery Act Transparency Statement|Sitemap