Transfer learning method and device
A transfer learning and sample technology, applied in the field of machine learning, can solve problems affecting system performance, sample quality deterioration, algorithm performance degradation, etc., to achieve the effect of improving sample quality and accuracy
- Summary
- Abstract
- Description
- Claims
- Application Information
AI Technical Summary
Problems solved by technology
Method used
Image
Examples
Embodiment 1
[0021] Such as figure 1 As shown, the transfer learning method of this embodiment includes steps S10-S40.
[0022] Step S10 is an initial step, in which parameters related to transfer learning are set and initialized. For example, set and initialize the input parameters of transfer learning, including labeled source distribution data L, unlabeled target distribution data U, and automatically labeled data sets TS of past cycles c = φ, the automatic labeling data set TS in the current cycle l = φ, the iteration period T for error detection, the total number of transfer learning iterations (referred to as the total number of iterations) K, the number of positive and negative samples automatically marked in each iteration p and q, the number of current iterations I, the error estimated in the past cycle Boundary ε pre , the error bound ε of the current cycle estimate next ,wait.
[0023] Step S20 is a sample acquisition step, that is, starting the migration learning iteration...
Embodiment 2
[0084] The migration learning method of this embodiment is basically the same as that of Embodiment 1, the difference is that in the cycle calculation step, Embodiment 1 uses the KNN graph model method based on statistics to calculate the error rate, while this embodiment uses the method based on cross-validation classification method. Specifically, the cycle calculation step of this embodiment includes: taking the automatically labeled data after each iteration as a sample, and dividing all samples in the current iteration cycle into at least two sets, one of which is used as a test set, and the remaining sets are used as The training set is calculated by using the cross-validation method to obtain the classification error probability of each sample, which is equivalent to the error rate of embodiment 1, and then according to the calculated classification error probability of each sample in the current iteration cycle, calculate the current The error bound of the iteration cy...
PUM
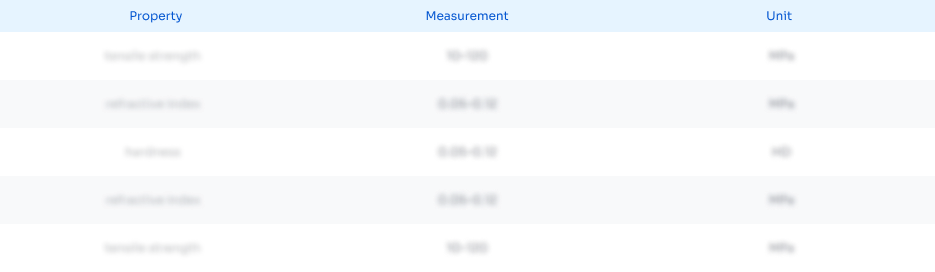
Abstract
Description
Claims
Application Information
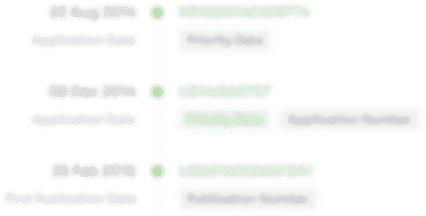
- R&D Engineer
- R&D Manager
- IP Professional
- Industry Leading Data Capabilities
- Powerful AI technology
- Patent DNA Extraction
Browse by: Latest US Patents, China's latest patents, Technical Efficacy Thesaurus, Application Domain, Technology Topic, Popular Technical Reports.
© 2024 PatSnap. All rights reserved.Legal|Privacy policy|Modern Slavery Act Transparency Statement|Sitemap|About US| Contact US: help@patsnap.com