Image pixel labeling method based on super pixel level features
A super pixel and image pixel technology, applied in image analysis, image data processing, instruments, etc., can solve the problems of slow labeling speed, labeling accuracy to be improved, large amount of nonlinear kernel function calculation, etc., to improve speed and reduce number , the effect of fast labeling speed
- Summary
- Abstract
- Description
- Claims
- Application Information
AI Technical Summary
Problems solved by technology
Method used
Image
Examples
Embodiment Construction
[0045] The embodiment of a method for labeling feature pixels based on the superpixel level proposed by the present invention is described in detail in conjunction with the accompanying drawings as follows: firstly, use the labeled training set images to pair the required feature encoder, linear SVM, and conditional random field parameters for the labeling process Carry out training, and then use the labeling module to label the image to be labeled. The labeling process is as follows figure 1 shown.
[0046] The steps to train the module are as follows:
[0047] (1) Extract the Dense-SIFT feature of each pixel of the training set image (that is, the SIFT feature with fixed scale and direction), where the scale is set to 1, the direction is set to 0, and the direction histogram window is set to 12×12. Since the calculation of each feature does not depend on other features, it can run in parallel, and a feature extraction method based on CUDA can be used.
[0048] Training enc...
PUM
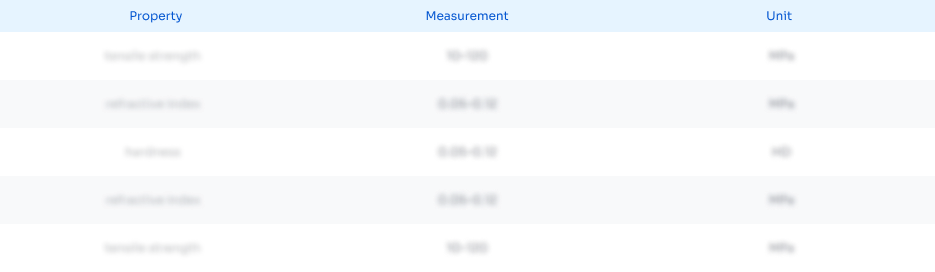
Abstract
Description
Claims
Application Information
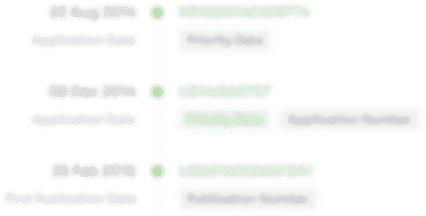
- R&D
- Intellectual Property
- Life Sciences
- Materials
- Tech Scout
- Unparalleled Data Quality
- Higher Quality Content
- 60% Fewer Hallucinations
Browse by: Latest US Patents, China's latest patents, Technical Efficacy Thesaurus, Application Domain, Technology Topic, Popular Technical Reports.
© 2025 PatSnap. All rights reserved.Legal|Privacy policy|Modern Slavery Act Transparency Statement|Sitemap|About US| Contact US: help@patsnap.com