Cloud platform decision forest classification method based on discrete weak correlation
A classification method and cloud platform technology, applied in the field of cloud computing, can solve problems such as unsatisfactory space efficiency and classification accuracy, reduce time and space overhead, improve quality, and enhance the ability to resist data noise.
- Summary
- Abstract
- Description
- Claims
- Application Information
AI Technical Summary
Problems solved by technology
Method used
Image
Examples
Embodiment Construction
[0018] The present invention will be further described below with reference to the accompanying drawings and embodiments.
[0019] A decision forest classification method for cloud platform based on discrete weak correlation ( figure 1 ), the method includes the following steps:
[0020] Step S1, generating a description file that makes the cloud platform decision forest optimal, and the description file includes the optimal total number of decision trees and the new dataset dataset of each decision tree;
[0021] The optimal total number of decision trees is obtained by the following method: multiply the number of Data_Node nodes of the Hadoop cloud platform by the number of Reduce tasks set uniformly by each node, and divide the obtained decision by 2 times the square root of the product obtained by m. The optimal total number of decision trees in the forest enables each Reduce task to calculate the entropy of an attribute independently after reduction; where m is the value...
PUM
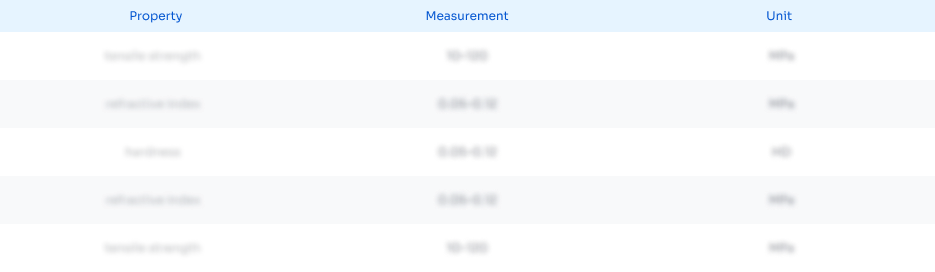
Abstract
Description
Claims
Application Information
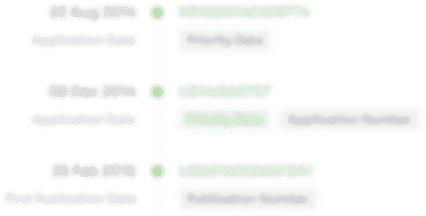
- R&D
- Intellectual Property
- Life Sciences
- Materials
- Tech Scout
- Unparalleled Data Quality
- Higher Quality Content
- 60% Fewer Hallucinations
Browse by: Latest US Patents, China's latest patents, Technical Efficacy Thesaurus, Application Domain, Technology Topic, Popular Technical Reports.
© 2025 PatSnap. All rights reserved.Legal|Privacy policy|Modern Slavery Act Transparency Statement|Sitemap|About US| Contact US: help@patsnap.com