Improved feature evaluation function based Bayesian spam filtering method
A technology for spam filtering and evaluating functions, applied in special data processing applications, electrical digital data processing, instruments, etc., can solve the problems of weak negative correlation performance, lack of performance of filtering methods, and different contribution capabilities of feature item category definition.
- Summary
- Abstract
- Description
- Claims
- Application Information
AI Technical Summary
Problems solved by technology
Method used
Image
Examples
Embodiment Construction
[0031] The Bayesian spam filtering method based on improved feature evaluation function is characterized in that the steps are as follows:
[0032] 1) Preprocess the training mail set: divide the mail into two sub-text sets S 1 ,S 2 , In which word segmentation is performed separately to form two feature item sets T 1 , T 2 ; 2) Respectively in two feature sets T 1 , T 2 Use the stop vocabulary table to delete prepositions, pronouns, adverbs, auxiliary words, conjunctions, and words whose frequency is lower than a given threshold p, and the processed feature item set is marked as T 1 ’, T 2 ’;
[0033] 3) Respectively in the feature item set T 1 ’, T 2 ’Uses an improved feature evaluation function to calculate the mutual information value MI(t k )’:
[0034] 3a) Set the feature vector set T = {t k ,k=1,2,...,n}, obtain the training set category set C={c in the network file text library j ,i=1,2,...,r};
[0035] 3b) Using formula (1) to calculate the correction coefficient λ:
[0036] ...
PUM
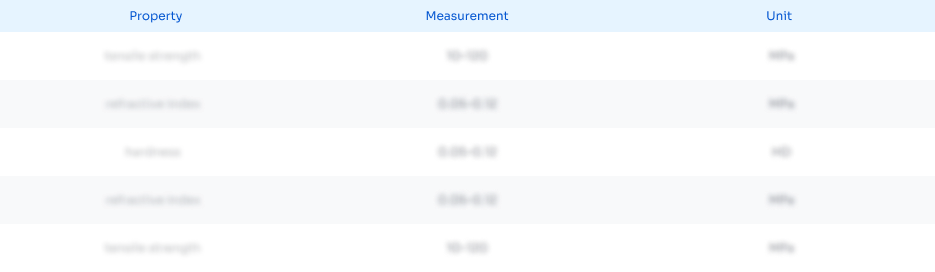
Abstract
Description
Claims
Application Information
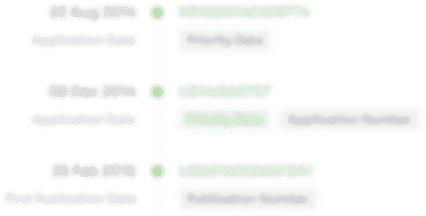
- R&D Engineer
- R&D Manager
- IP Professional
- Industry Leading Data Capabilities
- Powerful AI technology
- Patent DNA Extraction
Browse by: Latest US Patents, China's latest patents, Technical Efficacy Thesaurus, Application Domain, Technology Topic, Popular Technical Reports.
© 2024 PatSnap. All rights reserved.Legal|Privacy policy|Modern Slavery Act Transparency Statement|Sitemap|About US| Contact US: help@patsnap.com