Multi-level anomaly detection method based on exponential smoothing and integrated learning model
An exponential smoothing and integrated learning technology, applied in the field of integrated learning classification technology, can solve the problems of high false alarms, unsuitable detection, and low accuracy
- Summary
- Abstract
- Description
- Claims
- Application Information
AI Technical Summary
Problems solved by technology
Method used
Image
Examples
Embodiment Construction
[0066] In order to better understand the technical content of the present invention, specific embodiments are given together with the attached drawings for description as follows.
[0067] The flow chart of the multi-level anomaly detection method based on exponential smoothing, sliding window distribution statistics and integrated learning model of the present invention is as follows figure 1 shown.
[0068] Step 0 is the initial state of the abnormal detection method of the present invention;
[0069] During the statistical detection phase (steps 1-3):
[0070] Step 1 is to construct a set of key monitoring features based on application scenarios and domain knowledge;
[0071] Step 2 is to model each key feature (exponential smoothing or sliding window distribution statistics) according to the type of feature (discrete or continuous);
[0072] Step 3 is to monitor new records in real time. For each key feature of the new record, the type of feature is judged. The key feat...
PUM
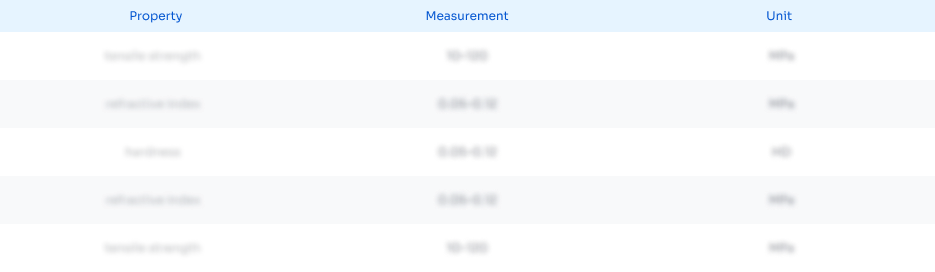
Abstract
Description
Claims
Application Information
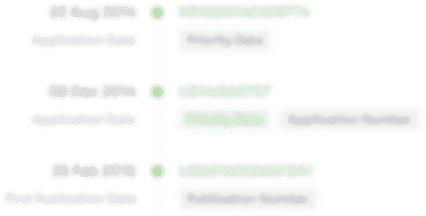
- R&D Engineer
- R&D Manager
- IP Professional
- Industry Leading Data Capabilities
- Powerful AI technology
- Patent DNA Extraction
Browse by: Latest US Patents, China's latest patents, Technical Efficacy Thesaurus, Application Domain, Technology Topic.
© 2024 PatSnap. All rights reserved.Legal|Privacy policy|Modern Slavery Act Transparency Statement|Sitemap