Multi-target evolutionary fuzzy rule classification method based on decomposition
A technology of multi-objective evolution and classification method, which is applied in the field of classification in data mining, and can solve problems such as difficult identification of minority classes
- Summary
- Abstract
- Description
- Claims
- Application Information
AI Technical Summary
Problems solved by technology
Method used
Image
Examples
Embodiment 1
[0051]Example 1: In recent years, with the continuous development of science and technology, people have a deeper understanding of the living space, and more and more problems encountered, more and more complex, in which the data classification problem is especially unbalanced data classification The problem is becoming more and more prominent in front of us. Unbalanced data classification is widely used in people's production and life, such as biomedical recognition, to obtain a certain data from yeast cells and determine whether the data comes from a certain internal part of yeast cells , the yeast cell has a total of 10 internal parts, the part to which the acquired data belongs has less data, and the remaining 9 internal parts have more data, which causes the imbalance between data, tumor In the detection, it is determined whether a person has breast cancer. In the crowd, the number of people who really have breast cancer is small, while the number of normal people without...
Embodiment 2
[0063] Embodiment 2: The multi-objective evolutionary fuzzy rule classification method based on decomposition is the same as embodiment 1, wherein step 4 initializes the population P formed by pop fuzzy classifiers and uses the fuzzy rule weight formula with weighting factors to determine the fuzzy rule weight w i ,i∈{1,...,pop}, in this example, initialize a population P consisting of 150 fuzzy classifiers and use the fuzzy rule weight formula with weighting factors to determine the fuzzy rule weight w i ,i∈{1,...,150} includes the following steps:
[0064] 4a. Randomly select a piece of data x from the training data set X rand =[x rand,1 ,...,x rand,n ,y rand ], corresponding to the piece of data x rand There is a fuzzy rule r rand =[rrand,1 ,...,r rand,n ,w rand , l rand ], where the fuzzy rule r rand The attribute values of the first n items are respectively recorded with the data x rand The fuzzy partition labels corresponding to the n attributes of , w rand ...
Embodiment 3
[0082] Embodiment 3: The decomposition-based multi-objective evolutionary fuzzy rule classification method is the same as that in Embodiment 1-2, wherein step 7 performs an evolutionary operation on the original population P and adopts the Chebyshev update method to update each chromosome in turn, and the result is composed of pop chromosomes The evolutionary population P′ of , in this example, the evolutionary population P′ composed of 150 chromosomes is obtained, including the following steps:
[0083] 7a. Chromosome Chromosome h ,h∈{1,...,pop} performs a single-point crossover operation to obtain two crossover daughter chromosomes chro h,cr_1 , chro h,cr_2 ,h∈{1,...,pop}, in this example, for chromosome chro h, h∈{1,...,150} performs a single-point crossover operation to obtain two crossover daughter chromosomes chro h,cr_1 , chro h,cr_2 ,h∈{1,...,150}.
[0084] 7b. Chromosomes of two crossed progeny h,cr_1 , chro h,cr_2 ,h∈{1,...,pop} is used as the mutated parent c...
PUM
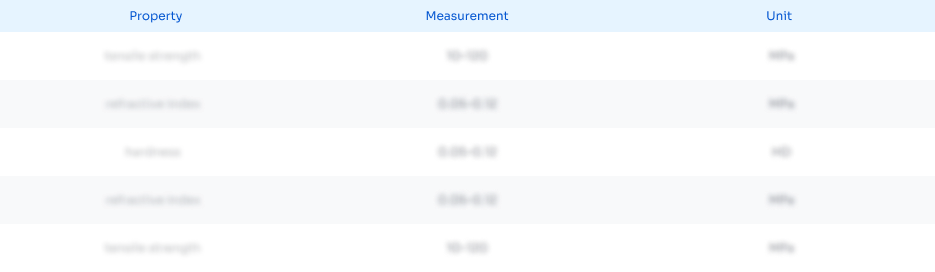
Abstract
Description
Claims
Application Information
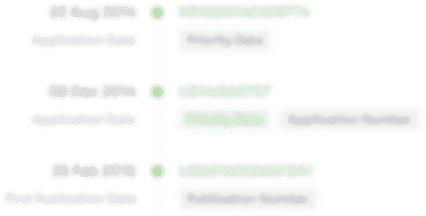
- R&D Engineer
- R&D Manager
- IP Professional
- Industry Leading Data Capabilities
- Powerful AI technology
- Patent DNA Extraction
Browse by: Latest US Patents, China's latest patents, Technical Efficacy Thesaurus, Application Domain, Technology Topic, Popular Technical Reports.
© 2024 PatSnap. All rights reserved.Legal|Privacy policy|Modern Slavery Act Transparency Statement|Sitemap|About US| Contact US: help@patsnap.com