Method for marking picture semantics based on Gauss mixture model
A Gaussian mixture model, semantic annotation technology, applied in character and pattern recognition, instruments, computer parts and other directions, can solve problems such as semantic gap
- Summary
- Abstract
- Description
- Claims
- Application Information
AI Technical Summary
Problems solved by technology
Method used
Image
Examples
Embodiment 1
[0095] Example 1: Partial examples of image segmentation, underlying feature extraction, and feature selection modules
[0096] Specific steps;
[0097] 1. Select a semantic concept from the provided image library, each semantic concept includes 200 images;
[0098] 2. Analyze the image color and quantify it.
[0099] 3. Carry out spatial segmentation on the color-quantized image to obtain a set of similar points of any shape, that is, the segmented image area.
[0100] 4. Extract color features, obtain 3-dimensional main color features, and the mean and variance of H, S, and V components in each image area, a total of 9-dimensional feature vectors.
[0101] 5. Extract texture features, obtain the mean and variance of Gabor wavelet coefficients in 4 scales and 6 directions, a total of 48 dimensional regional texture feature vectors.
[0102] 6. Integrate the image features and store them in the image feature library.
[0103] 7. Sampling the image feature library to genera...
Embodiment 2
[0107] Example 2: Partial examples of training
[0108] Specific steps such as Image 6 shown;
[0109] (1) Obtain the optimized image feature library according to the method of embodiment 1;
[0110] (2) Input the feature parameter extracted in (1) to the GMM trained, and seek its likelihood;
[0111] (3) the weighted summation of the matching probabilities of each characteristic parameter obtained in (2) to obtain the total matching degree Match;
[0112] (4) Compare the obtained Match with the threshold. If it is greater than the threshold, the contribution rate of each feature will be calculated; if it is less than the threshold, it will be counted and sent to the end judgment;
[0113] (5) Send back the feature whose Match in (4) is less than the threshold and does not meet the end condition for a new round of training; if the end condition is met, the training ends.
Embodiment 3
[0114] Embodiment 3: Some examples of semantic annotation:
[0115] The specific steps are shown in Figure 7;
[0116] (1) Obtain the optimized image feature library according to the method of embodiment 1;
[0117] (2) Input the feature parameters extracted in (1) into the trained GMM matrix to obtain the likelihood corresponding to each feature;
[0118] (3) According to the likelihood obtained in (2), obtain the probability of matching for each type of feature;
[0119] (4) The probability weighted summation corresponding to all features is used as the total matching Match;
[0120] (5) Compare the obtained Match with the threshold, if it is greater than the threshold, the contribution rate of each feature will be calculated; if it is less than the threshold, it will be counted and sent to the end judgment.
PUM
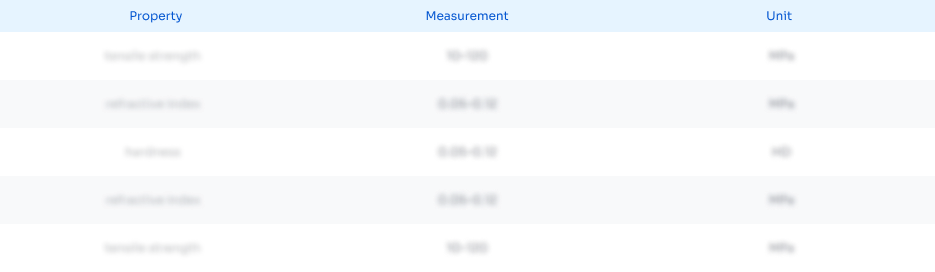
Abstract
Description
Claims
Application Information
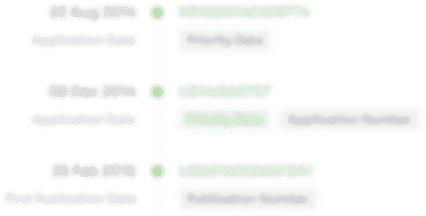
- R&D Engineer
- R&D Manager
- IP Professional
- Industry Leading Data Capabilities
- Powerful AI technology
- Patent DNA Extraction
Browse by: Latest US Patents, China's latest patents, Technical Efficacy Thesaurus, Application Domain, Technology Topic, Popular Technical Reports.
© 2024 PatSnap. All rights reserved.Legal|Privacy policy|Modern Slavery Act Transparency Statement|Sitemap|About US| Contact US: help@patsnap.com