A Method of Automatic Image Annotation Based on Semantic Scene Classification
An image automatic labeling and semantic scene technology, which is applied in the field of image automatic labeling algorithm based on semantic scene classification, can solve the problem of label hard classification without considering the mapping relationship between labels and semantic scenes, so as to improve the efficiency of the algorithm, improve the effect of labeling, The effect of reducing noise disturbance
- Summary
- Abstract
- Description
- Claims
- Application Information
AI Technical Summary
Problems solved by technology
Method used
Image
Examples
Embodiment Construction
[0055] The specific embodiments discussed are merely illustrative of implementations of the invention, and do not limit the scope of the invention. Embodiments of the present invention will be described in detail below in conjunction with the accompanying drawings.
[0056] The embodiment of the present invention in benchmark instance Iaprtc12 is as follows:
[0057] Symbolic representation: training set {X, Y}, test set {X′}, X represents the sample feature matrix, Y represents the label information of the training set, and X′ represents the test set feature matrix.
[0058] (1) Feature extraction
[0059] The five features of Gist (512D), DenseHue (100D), HarrisHue (100D), DenseSift (1000D) and HarrisSift (1000D) existing in the reference instance Iaprtc12 are used as the feature {X} of this embodiment.
[0060] (2) Scene detection example
[0061] According to the formula (1) and the label matrix Y to construct the label relationship graph C, according to the formula (2)...
PUM
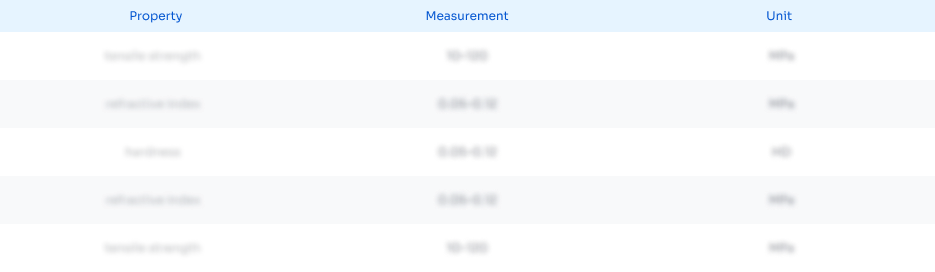
Abstract
Description
Claims
Application Information
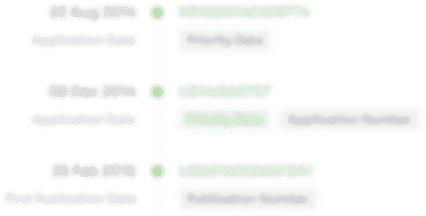
- R&D Engineer
- R&D Manager
- IP Professional
- Industry Leading Data Capabilities
- Powerful AI technology
- Patent DNA Extraction
Browse by: Latest US Patents, China's latest patents, Technical Efficacy Thesaurus, Application Domain, Technology Topic.
© 2024 PatSnap. All rights reserved.Legal|Privacy policy|Modern Slavery Act Transparency Statement|Sitemap