Method for establishing disease risk adjustment model
A technology for model establishment and disease risk, applied in the field of disease risk adjustment model establishment, which can solve problems such as falsely high cost treatment, biased judgment results, and increase model instability, so as to avoid overfitting and improve accuracy and reliability. Effect
- Summary
- Abstract
- Description
- Claims
- Application Information
AI Technical Summary
Problems solved by technology
Method used
Image
Examples
example 1
[0075] Example 1: Correlation analysis of predictors in a renal failure mortality model
[0076] Model #218: DRG 682,683,684
[0077] Data source: Memorial Herman Hospital, Texas Medical Center, USA
[0078] Number of patient samples: 2587 Discharge time 7 / 1 / 2004-6 / 30 / 2014
[0079] Number of significant variables screened out: 37
[0080] Number of strongly correlated variables: 4
[0081] Statistical method: Variance Inflation Factor (VIF)
[0082]
[0083] Note: The strong correlation is detected from the 37 variables screened out. The value below is the VIF value, and the variables with VIF>5 are marked.
example 2
[0084] Example 2: Importance List of Predictor Variables in a Mortality Model for Patients with Renal Failure
[0085] Model #218: DRG 682,683,684
[0086] Data source: Memorial Herman Hospital, Texas Medical Center, USA
[0087] Number of patient samples: 2587 Discharge time 7 / 1 / 2004-6 / 30 / 2014
[0088] Total number of model variables: 280
[0089] Number of significant variables screened out: 37
[0090] Statistical Methods: LASSO
[0091]
[0092] Note: The value below the variable represents the importance of the variable. The higher the value, the greater the influence of the variable on the model.
example 3
[0093] Example 3: Predictor selection for a renal failure mortality model
[0094] Model #218: DRG 682,683,684
[0095] Data source: Memorial Herman Hospital, Texas Medical Center, USA
[0096] Number of patient samples: 2587 Discharge time 7 / 1 / 2004-6 / 30 / 2014
[0097] Total number of model variables: 280
[0098] Number of significant variables screened out: 37
[0099] Statistical Methods: LASSO
[0100]
[0101]
[0102] NOTE: The variables rhabdomyolysis, chronic liver disease, and later were removed in conjunction with model and clinical experience; the strongly correlated variable - intra-organ tube was also removed.
PUM
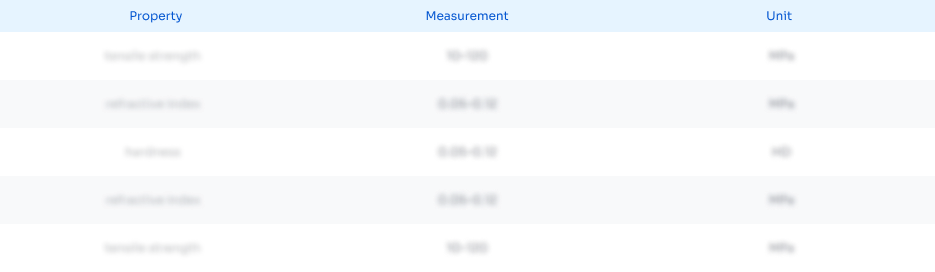
Abstract
Description
Claims
Application Information
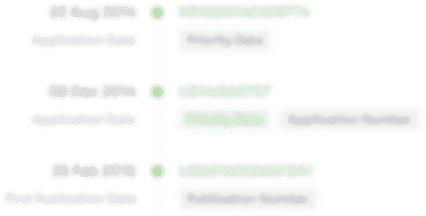
- R&D
- Intellectual Property
- Life Sciences
- Materials
- Tech Scout
- Unparalleled Data Quality
- Higher Quality Content
- 60% Fewer Hallucinations
Browse by: Latest US Patents, China's latest patents, Technical Efficacy Thesaurus, Application Domain, Technology Topic, Popular Technical Reports.
© 2025 PatSnap. All rights reserved.Legal|Privacy policy|Modern Slavery Act Transparency Statement|Sitemap|About US| Contact US: help@patsnap.com