Hyperspectral Remote Sensing Image Classification Method Based on Spatial Regularized Manifold Learning Algorithm
A technology of hyperspectral remote sensing and classification methods, applied in computing, computer parts, instruments, etc., can solve the problem of high computational complexity of limited and semi-supervised classification algorithms, and achieve the effect of improving the class separability
- Summary
- Abstract
- Description
- Claims
- Application Information
AI Technical Summary
Problems solved by technology
Method used
Image
Examples
Embodiment Construction
[0035] The present invention will be further described below in conjunction with the accompanying drawings and embodiments, but the protection scope of the present invention is not limited to the following embodiments.
[0036] The flow of the hyperspectral remote sensing image classification method based on the spatial regularization manifold learning algorithm provided by the present invention is as follows: figure 1 As shown, this method combines image segmentation and dimensionality reduction result alignment technology to maximize the role of spatial regular constraints to perform dimensionality reduction and classification of hyperspectral remote sensing images. The algorithm specifically includes the following steps:
[0037] (1), divide the hyperspectral remote sensing image X into n non-overlapping sub-blocks X i, i=1,...,n, where X represents hyperspectral remote sensing data, including N data points, and the dimension of each data point is D; X i Represents the set...
PUM
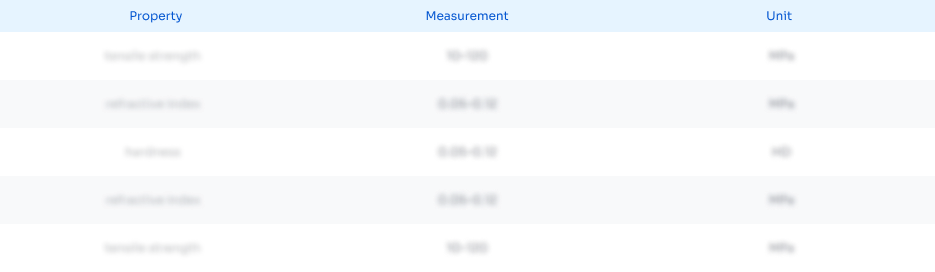
Abstract
Description
Claims
Application Information
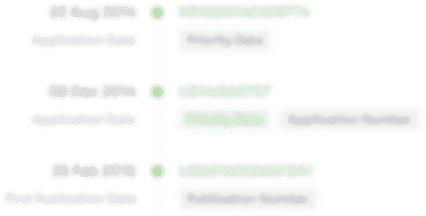
- R&D
- Intellectual Property
- Life Sciences
- Materials
- Tech Scout
- Unparalleled Data Quality
- Higher Quality Content
- 60% Fewer Hallucinations
Browse by: Latest US Patents, China's latest patents, Technical Efficacy Thesaurus, Application Domain, Technology Topic, Popular Technical Reports.
© 2025 PatSnap. All rights reserved.Legal|Privacy policy|Modern Slavery Act Transparency Statement|Sitemap|About US| Contact US: help@patsnap.com