A fast speaker identification method and system based on model growth clustering
A recognition method and model recognition technology, applied in speech analysis, instruments, etc., can solve the problems of long matching time and poor real-time performance, and achieve the effect of short matching time and good real-time performance
- Summary
- Abstract
- Description
- Claims
- Application Information
AI Technical Summary
Problems solved by technology
Method used
Image
Examples
Embodiment 1
[0070] like figure 1 As shown, a rapid speaker recognition method based on model growth clustering includes model training and model recognition;
[0071] Model training includes the following steps:
[0072] S1: Collect the voiceprint signals of multiple people including the speaker, that is, voice signals;
[0073] S2: Perform preprocessing and noise reduction processing on each voiceprint signal, and the preprocessing process sequentially includes pre-emphasis, framing, windowing and endpoint detection;
[0074] In the specific implementation process, in step S2, preprocessing each voiceprint signal specifically includes the following steps:
[0075] S2.1: Pre-emphasis, in the process of pre-emphasis, the voiceprint signal is moved to the appropriate frequency band through the filter,
[0076] The transfer function is: H(z)=1-0.9375z -1 ,
[0077] The resulting signal is:
[0078] S2.2: divided into frames, the voiceprint signal is divided into several frames at int...
Embodiment 2
[0114] like image 3 As shown, a rapid speaker recognition system based on model growth clustering includes: a client, a network connection module and a server, and the client and the server are connected through the network connection module;
[0115] Clients include:
[0116] Voiceprint acquisition module: used to collect the voiceprint signals of multiple people including speakers and output to the preprocessing module;
PUM
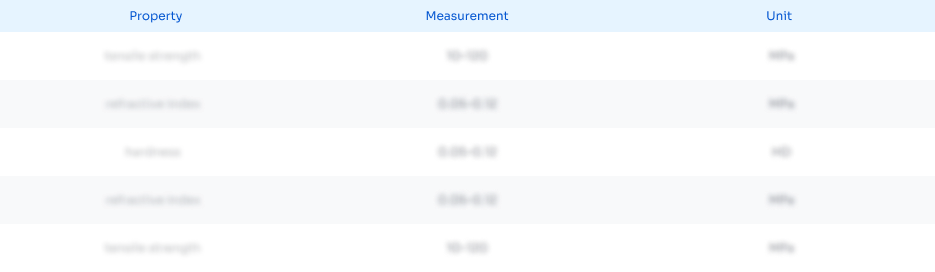
Abstract
Description
Claims
Application Information
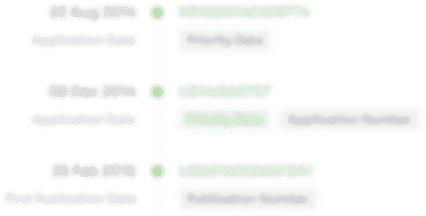
- R&D Engineer
- R&D Manager
- IP Professional
- Industry Leading Data Capabilities
- Powerful AI technology
- Patent DNA Extraction
Browse by: Latest US Patents, China's latest patents, Technical Efficacy Thesaurus, Application Domain, Technology Topic, Popular Technical Reports.
© 2024 PatSnap. All rights reserved.Legal|Privacy policy|Modern Slavery Act Transparency Statement|Sitemap|About US| Contact US: help@patsnap.com