RGB-D image classification method and system
A technology of RGB-D and RGB images, applied in the field of RGB-D image classification methods and systems, can solve the problems of low classification accuracy, insufficient classification of noisy data and high-similar images, and achieve strong representation ability and discrimination ability, classification Fast, overcoming the effect of noisy data
- Summary
- Abstract
- Description
- Claims
- Application Information
AI Technical Summary
Problems solved by technology
Method used
Image
Examples
Embodiment 1
[0055] Such as figure 1 Shown is a flow chart of a specific embodiment of an RGB-D image classification method of the present invention. see figure 1 , the concrete steps of a kind of RGB-D image classification method of this specific embodiment include;
[0056] S101. Process the source RGB image and the Depth image respectively to extract low-level features.
[0057] In this step, the low-level feature extraction of the image is to use a single-layer convolutional neural network (CNN) to perform convolution operations on the RGB image and the Depth image respectively to complete the extraction of low-level features of the image, such as figure 2 with 3 As shown, the specific process of extraction is as follows:
[0058] S1011. Use K filters for each size d i (such as d i =148) image is convolved, and the size of the convolution block is d p , normalize and whiten the convolutional blocks, and finally form K feature filter maps, each feature map has m convolutional bl...
Embodiment 2
[0091] On the basis of Embodiment 1, the present invention also provides an RGB-D image classification system. A kind of RGB-D image classification system of the present invention specifically comprises:
[0092] The low-level feature extraction module uses CNN to process the source RGB image and the Depth image to extract low-level features;
[0093] The middle-level feature extraction module, through the recurrent neural network (RNN), performs feedback learning on the low-level features of the image, and learns the middle-level features of the image;
[0094] The high-level feature extraction module is used to adopt the intra-block constraint dictionary learning method to perform feature group sparse representation of the middle-level features of the image, and obtain the high-level feature representation of the RGB-D image;
[0095] The classification module is used to input the high-level features of the RGB-D image into the linear support vector machine SVM to complete ...
PUM
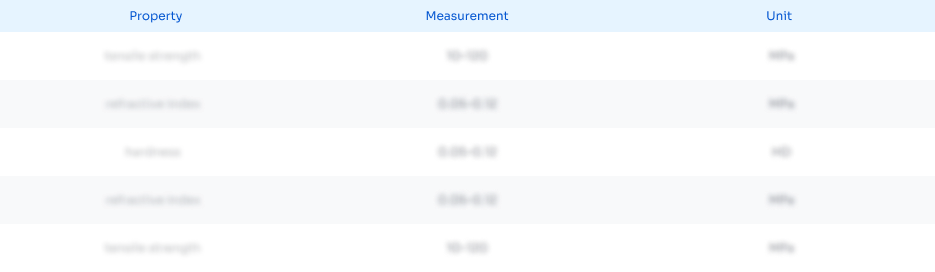
Abstract
Description
Claims
Application Information
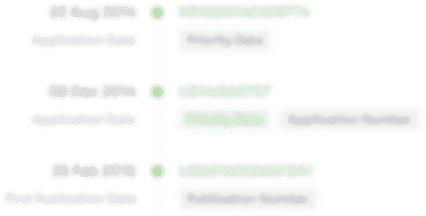
- R&D Engineer
- R&D Manager
- IP Professional
- Industry Leading Data Capabilities
- Powerful AI technology
- Patent DNA Extraction
Browse by: Latest US Patents, China's latest patents, Technical Efficacy Thesaurus, Application Domain, Technology Topic, Popular Technical Reports.
© 2024 PatSnap. All rights reserved.Legal|Privacy policy|Modern Slavery Act Transparency Statement|Sitemap|About US| Contact US: help@patsnap.com