Multi-stage nerve network model training method based on genetic algorithm
A neural network model and genetic algorithm technology, applied in the field of data mining, can solve the problem of input parameters affecting output in stages, and achieve the effect of excellent prediction effect
- Summary
- Abstract
- Description
- Claims
- Application Information
AI Technical Summary
Problems solved by technology
Method used
Image
Examples
Embodiment Construction
[0037] Embodiments of the present invention are described in further detail below in conjunction with the accompanying drawings:
[0038] A genetic algorithm-based multi-stage neural network model training method is implemented for the multi-stage neural network, and the multi-stage neural network is a neural network structure with multiple input layers. The multi-stage neural network system is mainly used in the case where the input parameters in engineering control have a chronological order, that is, the product processing process is different before and after, and the neural network structure with only one input layer cannot obtain accurate parameter control results. The quality of the neural network model is greatly affected by the initial weight and threshold. The genetic algorithm is used to select a set of reasonable initial weights for the neural network by virtue of its global search characteristics, so as to set the initial weight of the network as locally as possibl...
PUM
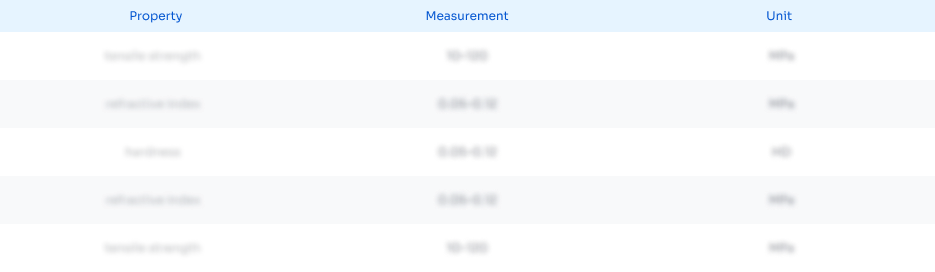
Abstract
Description
Claims
Application Information
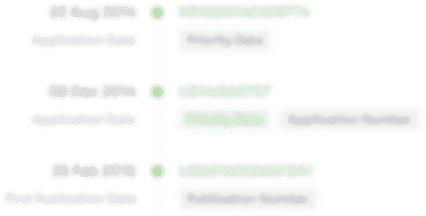
- R&D Engineer
- R&D Manager
- IP Professional
- Industry Leading Data Capabilities
- Powerful AI technology
- Patent DNA Extraction
Browse by: Latest US Patents, China's latest patents, Technical Efficacy Thesaurus, Application Domain, Technology Topic, Popular Technical Reports.
© 2024 PatSnap. All rights reserved.Legal|Privacy policy|Modern Slavery Act Transparency Statement|Sitemap|About US| Contact US: help@patsnap.com