Non local joint sparse representation based hyperspectral image super-resolution reconstruction method
A super-resolution reconstruction and hyperspectral image technology, applied in the field of hyperspectral image processing, can solve the problems of incomplete retention of structural features, insufficient spatial detail information, etc., achieve high definition and recognition, and improve the effect of visual quality
- Summary
- Abstract
- Description
- Claims
- Application Information
AI Technical Summary
Problems solved by technology
Method used
Image
Examples
Embodiment Construction
[0030] Now in conjunction with embodiment, accompanying drawing, the present invention will be further described:
[0031] Input: a low-resolution hyperspectral image Y and a panchromatic image P of the same scene.
[0032] 1. Training spectral dictionary
[0033] The input low spatial resolution hyperspectral image Y∈R m×n×L (m, n, L represent the image size), converted into a two-dimensional matrix form in Each column in represents a pixel vector of the hyperspectral image Y. use Train spectral dictionary D ∈ R L×K , K represents the number of atoms in the dictionary D, usually K is 2 to 3 times of L. The training steps are: Step 1: Initialize the dictionary D as Randomly selected K column elements, the intermediate quantity A=0, B=0, the maximum number of iterations T 1(Usually take 10-20).
[0034] Step 2: Right Each column element in Do the following:
[0035] 1) Use the minimum angle regression algorithm to solve the optimization problem get alpha i ...
PUM
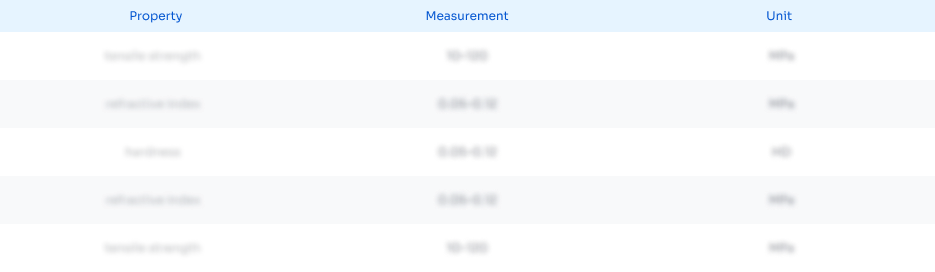
Abstract
Description
Claims
Application Information
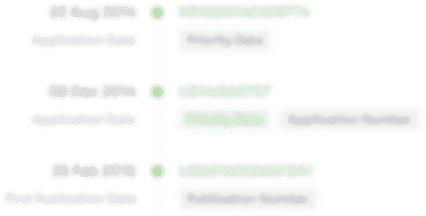
- R&D
- Intellectual Property
- Life Sciences
- Materials
- Tech Scout
- Unparalleled Data Quality
- Higher Quality Content
- 60% Fewer Hallucinations
Browse by: Latest US Patents, China's latest patents, Technical Efficacy Thesaurus, Application Domain, Technology Topic, Popular Technical Reports.
© 2025 PatSnap. All rights reserved.Legal|Privacy policy|Modern Slavery Act Transparency Statement|Sitemap|About US| Contact US: help@patsnap.com