Power system load prediction method using fuzzy decision-based neural network model
A neural network model, fuzzy decision-making technology, applied in fuzzy logic-based systems, biological neural network models, prediction and other directions, can solve problems such as unsatisfactory accuracy and large power load error
- Summary
- Abstract
- Description
- Claims
- Application Information
AI Technical Summary
Problems solved by technology
Method used
Image
Examples
Embodiment Construction
[0044] The present invention will be further described below in conjunction with the accompanying drawings. The following examples are only used to illustrate the technical solution of the present invention more clearly, but not to limit the protection scope of the present invention.
[0045] A neural network model power system load forecasting method based on fuzzy decision-making is characterized in that it comprises the following steps,
[0046] Step 1: Based on the neural network model of fuzzy decision-making, get the membership degree μ of all historical load samples i , to make a historical sample membership change curve.
[0047] Wherein step 1 includes the following steps:
[0048] Step 1.1: Calculate fuzzy positive and negative ideals Transform the given historical load data into triangular fuzzy numbers to obtain the matrix with Assuming that all indicators have equal weights, in Corresponding to n fuzzy index values, denoted as x...
PUM
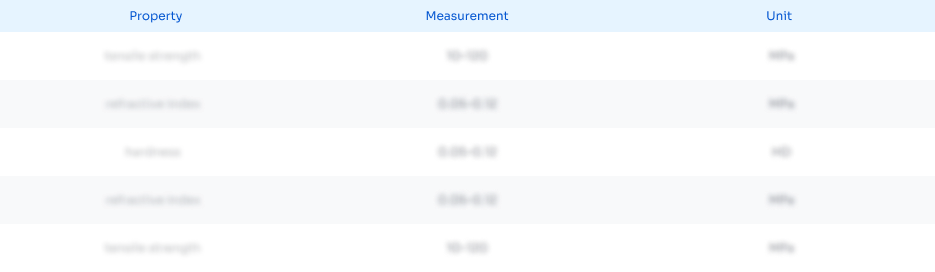
Abstract
Description
Claims
Application Information
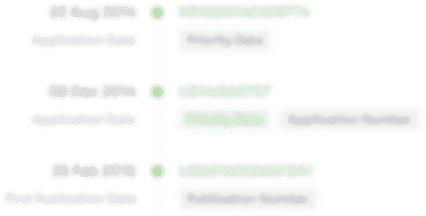
- R&D Engineer
- R&D Manager
- IP Professional
- Industry Leading Data Capabilities
- Powerful AI technology
- Patent DNA Extraction
Browse by: Latest US Patents, China's latest patents, Technical Efficacy Thesaurus, Application Domain, Technology Topic.
© 2024 PatSnap. All rights reserved.Legal|Privacy policy|Modern Slavery Act Transparency Statement|Sitemap