Short-term traffic flow prediction method based on convolutional neural network
A convolutional neural network and traffic flow technology, which is applied in the field of short-term traffic flow forecasting and can solve the problem of low forecasting accuracy.
- Summary
- Abstract
- Description
- Claims
- Application Information
AI Technical Summary
Problems solved by technology
Method used
Image
Examples
Embodiment Construction
[0054] The short-term traffic flow prediction method based on the convolutional neural network proposed by the present invention comprises the following steps:
[0055] Step 1) Preprocess the traffic data set to form a corresponding training set. The specific processing process is as follows:
[0056] Step 1.1) Use the historical traffic data of the predicted road section and its upstream and downstream sections in the data set to form an input matrix, and use the traffic data of the upstream section as the upper half of the input matrix, and the traffic data of the downstream section as the lower half of the input matrix, and predict the road section The traffic data is placed in the middle.
[0057] Step 1.2) Use the flow of the next time unit corresponding to each road segment in the input matrix as the expected output, and arrange the expected output according to the order of each road segment in the input matrix to form an output matrix.
[0058] Step 1.3) Normalize each...
PUM
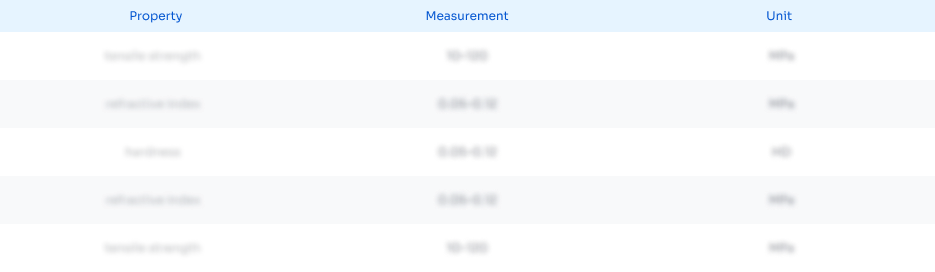
Abstract
Description
Claims
Application Information
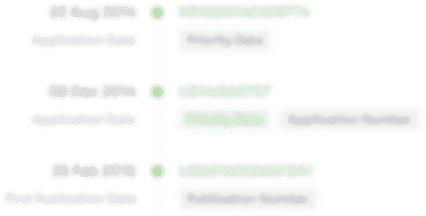
- R&D Engineer
- R&D Manager
- IP Professional
- Industry Leading Data Capabilities
- Powerful AI technology
- Patent DNA Extraction
Browse by: Latest US Patents, China's latest patents, Technical Efficacy Thesaurus, Application Domain, Technology Topic, Popular Technical Reports.
© 2024 PatSnap. All rights reserved.Legal|Privacy policy|Modern Slavery Act Transparency Statement|Sitemap|About US| Contact US: help@patsnap.com