Neighborhood rough set ensemble learning method based on attribute clustering
A technology of neighborhood rough set and integrated learning, which is applied in the direction of instruments, character and pattern recognition, computer components, etc., and can solve problems such as inability to deal with discrete data
- Summary
- Abstract
- Description
- Claims
- Application Information
AI Technical Summary
Problems solved by technology
Method used
Image
Examples
Embodiment Construction
[0039] Below in conjunction with accompanying drawing, the present invention will be further described:
[0040] A neighborhood rough set integrated learning method based on attribute clustering, including the following steps: First, in the data preprocessing stage, the data is normalized, and normalization refers to the linear transformation of the original data, so that the result value is mapped to [0-1]. Then, in the attribute clustering stage, by calculating the information gain of the attribute and selecting the attribute with a large information gain as the main attribute set, which is the center point of attribute clustering, and calculating the similarity between the remaining conditional attributes and the cluster center point, According to the similarity value, the attribute can be divided into several attribute clusters. Finally, in the base classifier ensemble training stage, first use the principle of neighborhood rough set to obtain the boundary domain of the t...
PUM
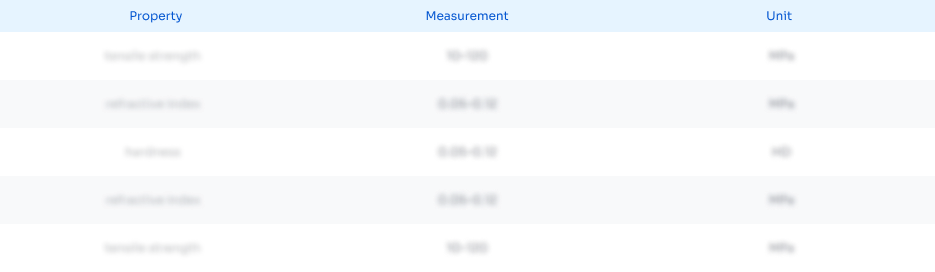
Abstract
Description
Claims
Application Information
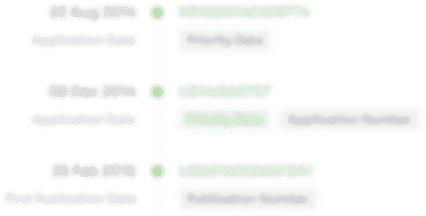
- R&D
- Intellectual Property
- Life Sciences
- Materials
- Tech Scout
- Unparalleled Data Quality
- Higher Quality Content
- 60% Fewer Hallucinations
Browse by: Latest US Patents, China's latest patents, Technical Efficacy Thesaurus, Application Domain, Technology Topic, Popular Technical Reports.
© 2025 PatSnap. All rights reserved.Legal|Privacy policy|Modern Slavery Act Transparency Statement|Sitemap|About US| Contact US: help@patsnap.com