Image Super-resolution Reconstruction Method Based on Maximum Linear Block Neighborhood Embedding
A neighborhood embedding and super-resolution technology, applied in the field of image processing, can solve problems such as unsuitable for engineering applications, difficulty in registration of multiple fuzzy and noisy images, and blurred images
- Summary
- Abstract
- Description
- Claims
- Application Information
AI Technical Summary
Problems solved by technology
Method used
Image
Examples
Embodiment 1
[0083] The present invention is innovating and researching in the field of super-resolution technology, specifically an image super-resolution reconstruction method based on maximum linear block neighborhood embedding, see figure 1 , the super-resolution reconstruction process of the present invention includes the following steps:
[0084] Step 1: According to the characteristics of the image to be reconstructed, construct a training sample set, extract mid- and high-frequency features from a set of training image blocks, and use this feature to perform hierarchical splitting clustering on the training set where the training samples are located. Clustering, the nonlinear manifold is approximately divided into multiple largest linear blocks, so that it is suitable for the use of linear embedding, so that the clustering results are more accurate. After clustering, according to the medium and high frequency characteristics, multiple largest linear blocks of different classes are c...
Embodiment 2
[0096] The image super-resolution reconstruction method based on the maximum linear block neighborhood embedding is the same as that in Embodiment 1. In step 1, the process of clustering and obtaining the maximum linear block is as follows:
[0097] (1a) When using the hierarchical splitting clustering method for clustering, the block with the largest degree of nonlinearity is selected from the training sample set X each time for splitting until all blocks reach the linearity of the set threshold, thus obtaining multiple clusters Class, called "maximum linear block", performs disjoint division on the training sample set X to obtain a set of disjoint maximum linear blocks X (i) , approximating multiple linear manifolds namely:
[0098]
[0099]
[0100]
[0101] where P is the number of local blocks, N i is the number of data points in the i-th local block, N is the total number of data points in all training sample sets X, and clustering is completed;
[0102] (1b) ...
Embodiment 3
[0125] The image super-resolution reconstruction method based on the maximum linear block neighborhood embedding is the same as that in Embodiment 1-2, and the process of classifying the low-resolution test image in step 2 is as follows:
[0126] (2a) Classify the input low-resolution image blocks, build a block histogram according to the pixel gradient information, and first calculate the gradient g(i, j) and angle θ(i, j) at a pixel point (i, j) j), where,
[0127] gradient:
[0128] Angle: θ(i,j)=arctan(g y (i,j) / g x (i,j)),0<θ(i,j)<2π;
[0129] (2b) For each test image patch, calculate its angle histogram h( ):
[0130] if g(i,j)>T g
[0131] where δ θ Represents the interval width in the histogram, in order to reduce the noise problem, use the threshold T g Used to limit the gradient amplitude;
[0132] (2c) Perform low-pass filtering on the obtained histogram to find the maximum value l 1 and the next largest value l 2 , judge whether the test image block i...
PUM
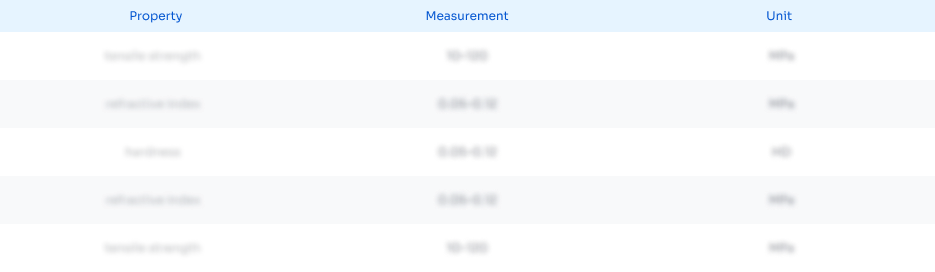
Abstract
Description
Claims
Application Information
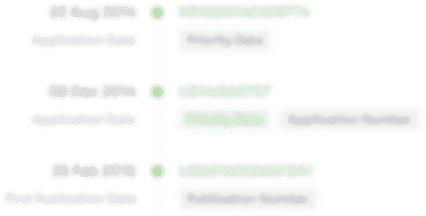
- R&D Engineer
- R&D Manager
- IP Professional
- Industry Leading Data Capabilities
- Powerful AI technology
- Patent DNA Extraction
Browse by: Latest US Patents, China's latest patents, Technical Efficacy Thesaurus, Application Domain, Technology Topic, Popular Technical Reports.
© 2024 PatSnap. All rights reserved.Legal|Privacy policy|Modern Slavery Act Transparency Statement|Sitemap|About US| Contact US: help@patsnap.com