Method for detecting riot and terror videos based on CNN and LSTM
A video detection and video technology, applied in character and pattern recognition, instruments, computer components, etc., can solve the problems of slow detection speed, high labor cost, limited detection accuracy and local characteristics, so as to avoid loss and ensure recognition The effect of precision
- Summary
- Abstract
- Description
- Claims
- Application Information
AI Technical Summary
Problems solved by technology
Method used
Image
Examples
Embodiment Construction
[0021] The present invention will be described in detail below in conjunction with the drawings and embodiments.
[0022] The present invention provides a method for detecting violent terror video based on CNN and LSTM, such as figure 1 As shown, the video detection method specifically includes the following steps:
[0023] The first step is to perform key frame sampling on the video to be detected and extract key frame features;
[0024] (1) For the video to be detected, first perform key frame sampling at equal intervals, with a sampling interval of 1 second, to obtain a key frame image.
[0025] (2) Downsample the key frame image to 227×227, input it into the CNN semantic model and the CNN scene model, and extract the CNN semantic feature and CNN scene feature of the key frame image respectively.
[0026] The CNN semantic feature and the CNN scene feature specifically include FC6 feature, FC7 feature and SPP feature respectively. Among them, FC6 feature and FC7 feature are commonly ...
PUM
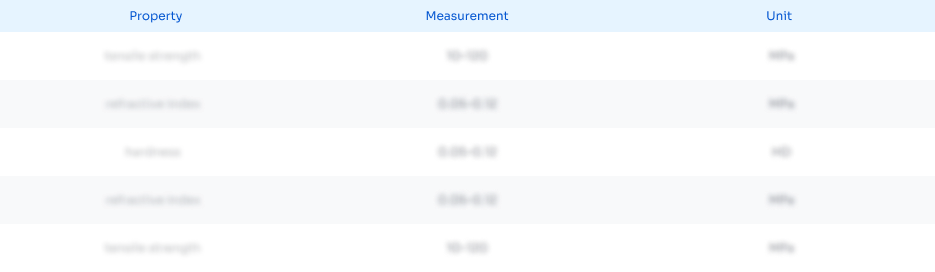
Abstract
Description
Claims
Application Information
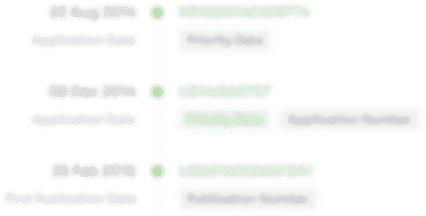
- R&D Engineer
- R&D Manager
- IP Professional
- Industry Leading Data Capabilities
- Powerful AI technology
- Patent DNA Extraction
Browse by: Latest US Patents, China's latest patents, Technical Efficacy Thesaurus, Application Domain, Technology Topic.
© 2024 PatSnap. All rights reserved.Legal|Privacy policy|Modern Slavery Act Transparency Statement|Sitemap