Parallel modular neural network-based byproduct gas real-time prediction method
A neural network and by-product gas technology, applied in the field of information, can solve problems such as affecting the balance adjustment of the gas system, affecting the production efficiency of enterprises, slow training speed, etc. The effect of prediction accuracy
- Summary
- Abstract
- Description
- Claims
- Application Information
AI Technical Summary
Problems solved by technology
Method used
Image
Examples
Embodiment Construction
[0025] The specific embodiments of the present invention will be described in detail below in combination with the summary of the invention and the accompanying drawings.
[0026] In order to better understand the technical scheme of the present invention, the following in conjunction with the attached figure 2 Embodiments of the present invention are described in detail, with figure 2 It is a pipe network structure diagram of a blast furnace gas system in a metallurgical enterprise. The four blast furnaces are the generating units of the gas system, and the production and manufacturing processes such as continuous casting, cold rolling, hot rolling, steel pipe factory, blooming, low-pressure boiler and power plant boiler are consumption units. . Real-time prediction of gas system operation status is crucial to the adjustment of gas system, so the present invention proposes a parallel modular neural network prediction method to realize real-time prediction of production and...
PUM
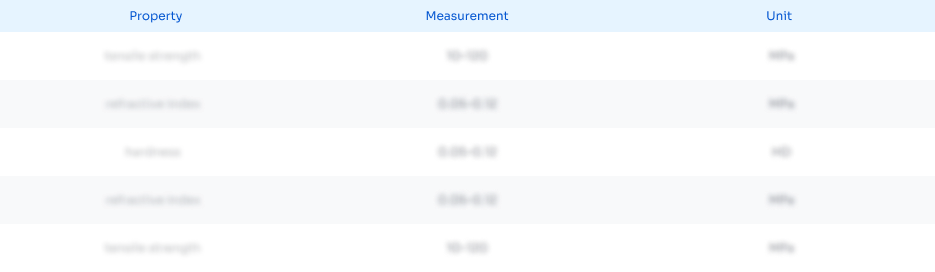
Abstract
Description
Claims
Application Information
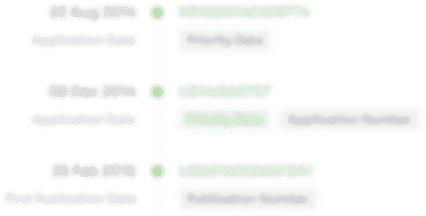
- R&D Engineer
- R&D Manager
- IP Professional
- Industry Leading Data Capabilities
- Powerful AI technology
- Patent DNA Extraction
Browse by: Latest US Patents, China's latest patents, Technical Efficacy Thesaurus, Application Domain, Technology Topic, Popular Technical Reports.
© 2024 PatSnap. All rights reserved.Legal|Privacy policy|Modern Slavery Act Transparency Statement|Sitemap|About US| Contact US: help@patsnap.com