Front vehicle parameter identification method based on multitask convolution nerve network
A convolutional neural network, convolutional neural technology, applied in neural learning methods, biological neural network models, character and pattern recognition, etc. To solve problems such as simplification, to achieve the effect of strong anti-environmental interference ability, strong scalability, and enhanced predictability
- Summary
- Abstract
- Description
- Claims
- Application Information
AI Technical Summary
Problems solved by technology
Method used
Image
Examples
Embodiment Construction
[0035] The specific embodiments of the present invention will be described in detail below in conjunction with the technical solutions and accompanying drawings. as attached figure 1 As shown, a vehicle parameter identification method based on multi-task convolutional neural network, including the following steps:
[0036] A. Design and training of convolutional neural network structure
[0037] A1. Convolutional neural network is a weight-sharing multi-layer neural network based on deep learning theory. The input layer of the convolutional neural network is an RGB-D image, and the size of the image pixel value is 106×106. In order to correct the uneven illumination in the scene, highlight the edge features of the image, and speed up the rapid convergence of the convolutional neural network training, the input image W is preprocessed with local contrast normalization (LCN), and its general expression is:
[0038] I ^ ( ...
PUM
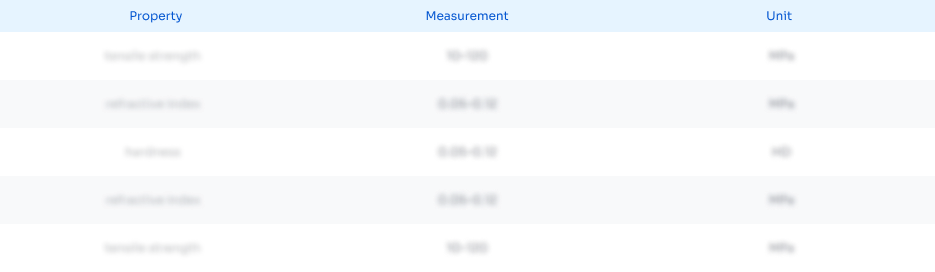
Abstract
Description
Claims
Application Information
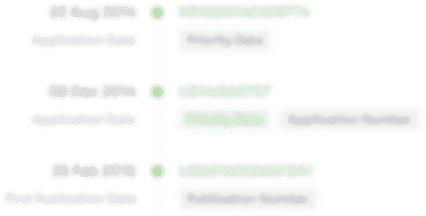
- R&D Engineer
- R&D Manager
- IP Professional
- Industry Leading Data Capabilities
- Powerful AI technology
- Patent DNA Extraction
Browse by: Latest US Patents, China's latest patents, Technical Efficacy Thesaurus, Application Domain, Technology Topic.
© 2024 PatSnap. All rights reserved.Legal|Privacy policy|Modern Slavery Act Transparency Statement|Sitemap