Scene identification method based on deconvolution deep network learning with weight
A deep network and scene recognition technology, applied in the field of scene recognition of deconvolution deep network learning, can solve problems such as hindering classification accuracy, confusion of residential areas in terminal buildings, and low classification accuracy
- Summary
- Abstract
- Description
- Claims
- Application Information
AI Technical Summary
Problems solved by technology
Method used
Image
Examples
Embodiment Construction
[0062] see figure 1 , the present invention provides a kind of scene recognition method based on deconvolution depth network learning with weight, it comprises the following steps:
[0063] 1) Construct a weighted deconvolution deep network model, use the weighted deconvolution deep network model to learn the original input image, and obtain the feature maps on different scales of each image;
[0064] 1.1) Construct a weighted deconvolution deep network model:
[0065] C ( l ) = λ l 2 | | y ^ l - y | | 2 2 + Σ k = 1 K ...
PUM
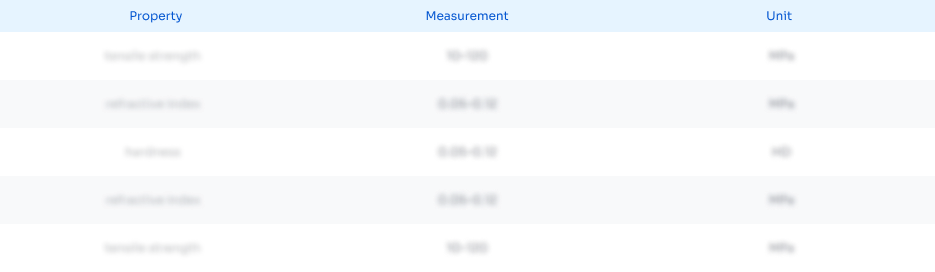
Abstract
Description
Claims
Application Information
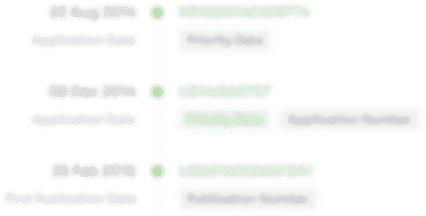
- R&D Engineer
- R&D Manager
- IP Professional
- Industry Leading Data Capabilities
- Powerful AI technology
- Patent DNA Extraction
Browse by: Latest US Patents, China's latest patents, Technical Efficacy Thesaurus, Application Domain, Technology Topic, Popular Technical Reports.
© 2024 PatSnap. All rights reserved.Legal|Privacy policy|Modern Slavery Act Transparency Statement|Sitemap|About US| Contact US: help@patsnap.com