Method for establishing probability matrix decomposition model based on node user
A technique of probabilistic matrix decomposition and construction method, applied in the field of data mining, can solve problems such as difficulties in obtaining potential information, and achieve the effect of improving prediction accuracy
- Summary
- Abstract
- Description
- Claims
- Application Information
AI Technical Summary
Problems solved by technology
Method used
Image
Examples
Embodiment Construction
[0020] The technical solution of the present invention will be described in further detail below in conjunction with the accompanying drawings and specific embodiments.
[0021] The present invention proposes a probability matrix decomposition model based on node users, figure 1 It is an overall schematic diagram of the present invention, including:
[0022] Step 1: Define the user's "influence", and define the user's "influence" according to the influence of the social network on the recommendation, that is, use the social relationship matrix to measure the user's "influence" in the recommendation system, let T∈{0 ,1} n*n Indicates the social relationship matrix, 1 means there is a relationship, 0 means no relationship, the more nodes with relationships, the greater the influence of the node; G=(V,E) means the network topology, n=|v| means the node number, v i represents node i, e ij represents an edge between nodes i and j.
[0023] Step 2: Formal measurement of node us...
PUM
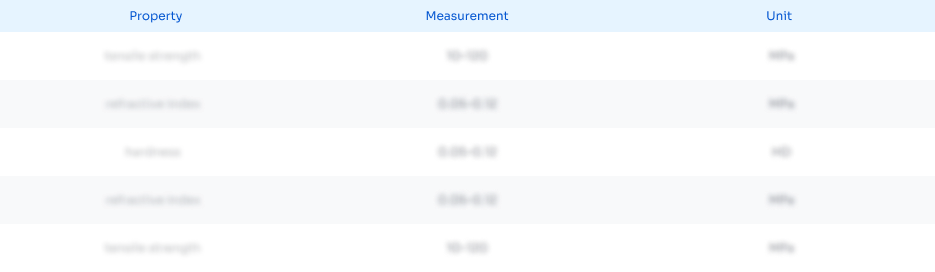
Abstract
Description
Claims
Application Information
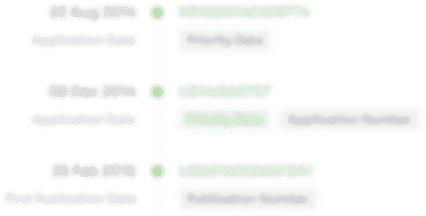
- R&D Engineer
- R&D Manager
- IP Professional
- Industry Leading Data Capabilities
- Powerful AI technology
- Patent DNA Extraction
Browse by: Latest US Patents, China's latest patents, Technical Efficacy Thesaurus, Application Domain, Technology Topic, Popular Technical Reports.
© 2024 PatSnap. All rights reserved.Legal|Privacy policy|Modern Slavery Act Transparency Statement|Sitemap|About US| Contact US: help@patsnap.com