Image semantic annotation method based on super pixel segmentation
A technology of superpixel segmentation and semantic annotation, applied in the field of image semantic analysis based on superpixel segmentation and convolutional neural network, can solve the problem of image annotation that cannot be universally solved, and achieves improved accuracy and robustness. The effect of improving computational efficiency and simplifying complexity
- Summary
- Abstract
- Description
- Claims
- Application Information
AI Technical Summary
Problems solved by technology
Method used
Image
Examples
Embodiment Construction
[0020] As shown in the figure, an image semantic annotation system based on superpixel segmentation, the semantic annotation system is divided into two parts: the first part is the superpixel block feature extraction part. The first part involves converting multi-level superpixel blocks into feature image blocks that can be input into the convolutional neural network for training, and for each superpixel block, it needs to be expanded with the geometric features of the superpixel, and requires A support vector machine is used to weight the features of the superpixel block. In the second part, the multi-level super-pixel features are integrated into the pixel level, and the pixel-level conditional random field model is established, and the reasoning is carried out through the idea of great a posteriori margin, and the image can be obtained by solving the model Labeled results. The technical problem to be solved by the present invention is to provide an image semantic annot...
PUM
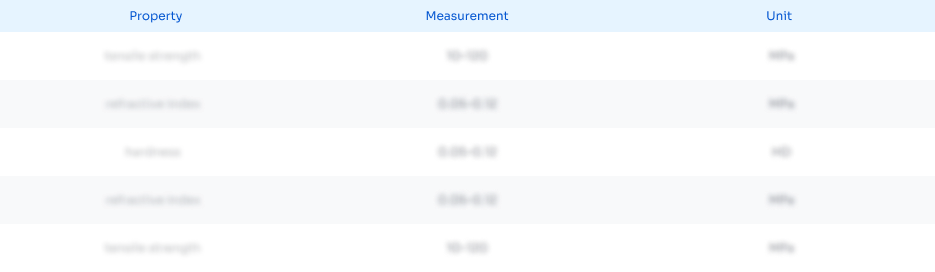
Abstract
Description
Claims
Application Information
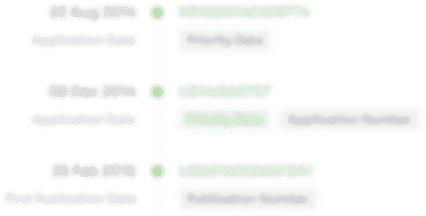
- R&D Engineer
- R&D Manager
- IP Professional
- Industry Leading Data Capabilities
- Powerful AI technology
- Patent DNA Extraction
Browse by: Latest US Patents, China's latest patents, Technical Efficacy Thesaurus, Application Domain, Technology Topic, Popular Technical Reports.
© 2024 PatSnap. All rights reserved.Legal|Privacy policy|Modern Slavery Act Transparency Statement|Sitemap|About US| Contact US: help@patsnap.com